J. MERILAHTI1, I. KORHONEN2
1. VTT Technical Research Centre of Finland, researcher at Digital Health group; 2. Information Technologies for Healthcare at TUT Tampere University of Technology.
Corresponding author: Juho Merilahti, VTT Technical Research Centre of Finland, researcher at Digital Health group, Kaitoväylä 1, 90570, P.O. Box 1100, Oulu, Finland, Tel: +358 40 7781 104, Fax: +358 20 722 3380, E-mail: Juho.Merilahti@vtt.fi
Abstract
Background: Physical functioning is a key factor in independent living, and its preclinical state assessment and monitoring during the subject’s normal life would be beneficial. Objectives: The aim of the study is to analyse associations between ambulatory measured physical activity behaviour and sleep patterns (wrist actigraphy) and self-reported difficulties in performing activities of daily living. Participants, setting and design: 36 residents in assisted living facilities and nursing homes (average age=80.4±9.0 years) without dementia in free living conditions participated. Actigraphic monitoring is integrated with the facilities’ social alarm system. Measurements: Indices on activity level, activity rhythm, sleep pattern and external stimuli response of sleep-wake behaviours were extracted from the actigraph data and correlated (Spearman rank-order correlation) with activities of daily living measures. Bonferroni correction for multiple comparisons was applied. Results: Activity level (ρ=-0.49, p<0.05) and night-time activity variance (ρ=-0.69, p<0.01) had correlation with the activities of daily living scores. The similarity of subject-wise activity pattern to facility common activities had a trend with activities of daily living (ρ=-0.44, p<0.1). In longitudinal case analysis, sleep and activity patterns were found to be associated with local weather variables. Conclusions: Activity patterns as measured by actigraphy may provide objective information on older people’s behaviour related to functioning state and its changes in nursing home and assisted living facility settings. However, variance between individuals was large in this dataset which decreases the reliability of the results. Furthermore, external stimuli such as weather and facility-related activities can affect subjects’ activity and sleep behaviour and should be considered in the related studies as well.
Key words: Activities of daily living, sleep, circadian rhythm, actigraphy, nursing home, assisted living.
Introduction
Physical functioning, describing a person’s ability to manage day-to-day activities independently or assisted, varies at different stages of health, life situations, and age (1). In addition to the physical capabilities, functioning is affected by environmental and personal factors (2). If functioning status is maintained at a higher level than minimally required by the surroundings, it can delay and shorten the period of aid needed and thus could lengthen the time of independence. Hence, assessment and follow-up of the preclinical state of functioning (before disabilities occur) for a person potentially at risk (typically an older adult) should be given focus and studied further.
Different low-cost technologies, such as pedometers, fitness trackers, or even novel computer controllers, enable the recording of a subject’s activity behaviour during normal life, which further could provide means to monitor the user’s health or functioning status. These technologies could “be validated against a battery of currently used—and widely accepted—techniques and indices” (3) such as physical functioning estimates.
The actigraphy, being one example of such a technology, is a widely used modality measuring activity and rest behaviour during daily life. The actigraph unit is typically worn on the wrist, which also makes it reasonably unobtrusive to wear for extended periods. It is typically used in sleep research and sleep medicine to infer user’s activity levels, circadian rhythm parameters, and sleep/wake patterns (4). There are recommendations that suggest the usefulness of actigraphy in sleep and circadian rhythm monitoring among older people, and especially among nursing home residents (4, 5). Actigraphy is also reported to provide meaningful information on the behaviour patterns associated with the functioning state of older adults (6-9). Thus it could be a valuable instrument to assess and monitor the preclinical risk of deteriorated functioning and disabilities in this older adult population.
Some studies already exist on the topic. For example changes in functioning and health have been associated with changes in sleep patterns. Short and long sleepers are more likely to have functional limitations among community-dwelling older people (7, 10). Longer daytime naps and disrupted sleep have also been associated with functional limitations (11).
Human biological daily rhythms (circadian rhythms) are stimulated by environmental factors such as bright light, social activity, food, and physical exercise (12). It has been suggested that the ability to maintain a regular endogenous rhythm is attenuated as we age (13), and monitoring of these rhythms might as well give indication of the functioning status changes.
For example later diurnal activity timing and activity level were associated with impaired functioning (instrumental activities of daily living; IADL) among community-dwelling older adults (8). Dissimilarity between the daily activity patterns tends to indicate a worsening condition and vice versa (14, 15). A similar trend was found for women with dementia, when compared with functioning and health instruments’ scores (9). Also more fragmented daily activity patterns were associated with worse functioning status.
Since functioning and ageing might influence on an older person’s ability to respond to the different environmental cues and their disruptions (13), measures on these stimulus-response associations could as well be used when evaluating the person’s functioning status. Associations have been encountered between physical activity and weather conditions such as daily temperature, day length, and sunshine amount for community-dwelling older adults (16, 17). However, physical activity correlations with weather were not significantly explained by physical functioning status for these subjects (17). We did not encounter studies that report results of sleep pattern responses to weather variables and how the responses would be affected by the subject’s physical functioning status.
The objective was to study if rest and activity patterns measured by an actigraph (wrist-worn) in daily living are associated with the self-reported functioning status of nursing home and assisted living facility residents without dementia, when actigraph data were collected in free living conditions. In addition, we study how the activity patterns’ response to environmental stimuli (facility activity rhythm) is associated with the self-reported functioning status. We also present two cases where changes in the activity and sleep patterns can be associated with the changes in functioning over time.
Methods
Material and subjects
The study material was collected from two nursing homes and from two assisted living facilities in Finland. The facilities were selected because the actigraph system was already installed there, and the residents and the personnel were familiar with the system. The inclusion criteria were willingness to participate in the study. The exclusion criteria were chronic disease seriously affecting wrist movements, having an acute disease, or having a disturbing event such as surgery during the recording. All the subjects gave a written consent. The study was accepted by the Regional Ethics Committee of the Northern Ostrobothnia Hospital District. For the analysis, we excluded the data from persons with dementia, since the actigraphy data can differ between people with and without dementia (6), possibly due to the disrupted sleep-wake cycle related to dementia. The dementia criterion was set at the Mini Mental State Examination (MMSE) score of below 21 and the Clinical Dementia Rating (CDR) score of above 0.5. Actigraph data and functioning measures from 36 subjects were included in the cross-sectional analysis (29 females, average age 80.4 years with standard deviation (SD) of 9.0 years, MMSE average score of 26.7 with SD of 3.0). Since the actigraph system stores the data on the facility’s server by default, we were able to access very long recordings for seven subjects. From these, two representative recordings were selected for presentation.
Health and functioning measures
The health and functioning status was assessed with a questionnaire on activities of daily living. The questionnaire included 14 items on a four-point scale (18). The grades describe the difficulty in performing an activity (without difficulty=1 / with difficulty, but without help=2 / only with help=3 / not able to perform=4). Subjects were asked to evaluate whether they were able to move outdoors, walk between rooms, use stairs, walk at least 400 meters, carry a heavy object, use the toilet, wash themselves, dress and undress, get in and out of the bed, prepare food, feed themselves, cut their toenails, do light housework, and do heavy housework. We have used the sum of all the components’ scores to describe the functioning status of a subject in the analysis. Similar questionnaires are reported to have 0.85-1.0 inter-rater reliability and 0.8-0.99 test-retest correlations (19). The ADL questionnaire has very similar content with the widely used Katz Index (ADL) and Brody scale (IADL). Experienced researchers collected the data using face-to-face interviews.
Equipment (actigraphy)
The actigraph device in the study is a wrist-worn online activity monitor (IST WristCare, Vivago, Helsinki, Finland; www.vivago.fi, telemetric actigraph), which can observe a person’s minute-to-minute activity level and sleep/wake patterns continuously (20). The sensing modality in the device detects micro- and macro-movement of the wrist, which is transformed to an activity epoch value for each minute (in arbitrary units). In addition, the device monitors wearing adherence (detection of whether the device is on the wrist) based on skin conductivity measurement (20). This helped care personnel to remind the subjects to wear the device if they forgot to put it on after a removal (for example due to shower or sauna). The telemetric actigraph’s primary purpose of use is as a social alarm device (panic button on a wrist-worn device). The system is reported to perform sleep/wake classification with a similar accuracy to a traditional actigraph when compared with polysomnography (the golden standard in sleep research) (21). In addition, it is found to be highly sensitive in detecting self-reported naps among older adults (21). During the study, the minute-to-minute activity data were stored automatically on an institute’s central computer, from which the data were extracted and anonymised before the analysis.
Actigraphy data analysis procedures
Most commonly, actigraphy is used to record a user’s activity levels, circadian rhythm parameters, and sleep/wake patterns objectively (5). Since there is a vast number of processing methodologies targeted at actigraphy data, we intended to select the most typically reported parameters for quantifying sleep and activity rhythm patterns in studies with similar objectives to ours. A new analysis method for describing an activity behaviour response to an environmental stimulus (in this case, a facility’s joint activities, such as meals and social events) was created. With the measure, we intend to study a stimuli-response relationship with the health status of older people (13). In addition, a manufacturer-specific parameter for describing diurnal activity rhythm strength was selected in the analysis. Since the reliability of the wrist-worn actigraphy data analysis procedures is reported to benefit even from two weeks’ measurement (22), we targeted data samples of 14 days in length, but accepted recordings of at least 7 days in length.
The following procedures and parameters were included in the analysis:
An estimation of activity pattern’s fit on 24-hour sinusoidal wave (the procedure fits a single 24-hour cosinor function with an actigraphy minute-to-minute data). The analysis results values for mesor (MESOR, mean of the activity data), amplitude (AMPL, difference between mesor and the peak of the fitted waveform), and acrophase (PHASE, timing of the activity rhythm for example peak at 15:48 that is 3:48 PM) (23). MESOR and AMPL are in the device specific activity units.
The 24-hour autocorrelation (AUTOCORR) describes the similarity of the activity rhythm (24) between days, and was selected because the physical activity is assumed to vary with a 24-hour rhythm. In practice, the analysis compares the actigraphy time series data to itself with a 24-hour delay (1440 minutes). The values vary between one for perfectly matching data, and minus one when the phase (that is the daily activity timing) is exactly the opposite, while a value close to zero indicates a large day-to-day variation in activity patterns.
The circadian rhythm parameters, inter-daily stability (IS), intra-daily variability (IV), and relative amplitude (RA) are frequently reported in the studies including a similar type of subject as in the current analysis. IS describes the stability between the days, implying a stable activity rhythm. IS values vary between one for a perfect match between the days and zero for very arrhythmic activity behaviours. IV quantifies the fragmentation of the rhythm and activity. IV gets small values for sine-wave-like data and values close to two for highly disrupted activity patterns. For example, it was reported that a high IV value (>1) was an indication of daytime naps and/or night-time awakenings (25). RA is the normalised ratio between the most active 10 hours and the least active 5-hour periods (13, 14). The most significant difference for IV, IS and RA calculations compared to the other utilized procedures is that the minute-to-minute activity data is at first converted to present the active minutes count for each hour (14). For the telemetric actigraph, minute-epochs with a value of four or above were categorized as active. The threshold selection was based on a concurrent validation analysis between traditional (Actiwatch, Cambridge Neurotechnology in 2003) and telemetric actigraph (data not shown). The selected threshold yielded the strongest correlation and the smallest error between these two recording devices for the IV, IS and RA parameters. A more detailed description of the calculation of the IV, IS and RA parameters is described in related research (14).
Total sleep time (TST), daytime sleep/passivity amount (NAP), sleep efficiency (SE, percentage of sleep when in bed), and number of awakenings (AWAKN) and night-time activity deviation (NIGHT ACT) present sleep patterns indices in the analysis. TST and NAP are based on the minute-to-minute sleep/wake classifications provided by the telemetric actigraph (typical output of an actigraph device). The subjects or the nursing personnel wrote down the bed times (going to sleep and getting out of bed) during the study, which were used in the calculation of TST and SE. A single researcher visually observed the quality of sleep log markings and removed potential false values. NAP is a sum of sleep detections during the daytime between 9 am and 9 pm. The same daytime period was used in related research (6). Awakenings (AWAKN) were counted by detecting the transition from sleep to wake when in bed. NIGHT ACT is the standard deviation of the activity signal while in bed, and it is supposed to provide information about sleep quality/restlessness (26). A manufacturer-specific parameter describing circadian rhythm strength (CRS) was calculated by dividing night-time activity (11 pm to 5 am) with the activity of the previous day (8 am to 8 pm) (27).
A new indicator was created to describe how much a personal activity correlates with an institute’s joint activity (named housing correlation (HOUSE)). The indicator is formed with a following procedure:
1) A period close to ADL inquiry which included 14 days of actigraph data for the subject of interest and which included actigraph data from other residents without a major interruption was identified. 2) The actigraph data from each subject were normalized and smoothened (scaled from zero to one and filtered with a 60-minute moving average). This helps to reduce differences between the subjects’ mean activity levels and better synchronises the timing of the common activities between the individuals. 3) These normalized actigraph data are averaged for each minute over all the residents (excluding the person of interest). This combined 14-day long activity data is called a grand average. 4) From the grand average common, facility-specific active periods are identified by subtracting the mean of the daytime grand average activity for each day and preserving only positive values (the timestamps of the daytime were identified from the grand average for each day individually using ad hoc threshold values). This residual data were considered to represent facility activities such as meals or social events (see Fig. 1). 5) The Pearson correlation was calculated for each subject, between the individual’s actigraph data and the grand average-based facility activities data.
This procedure forms the HOUSE indicator that is close to 1 for perfect synchrony between the facility activities and the individual’s activity, and close to zero for poor synchrony.
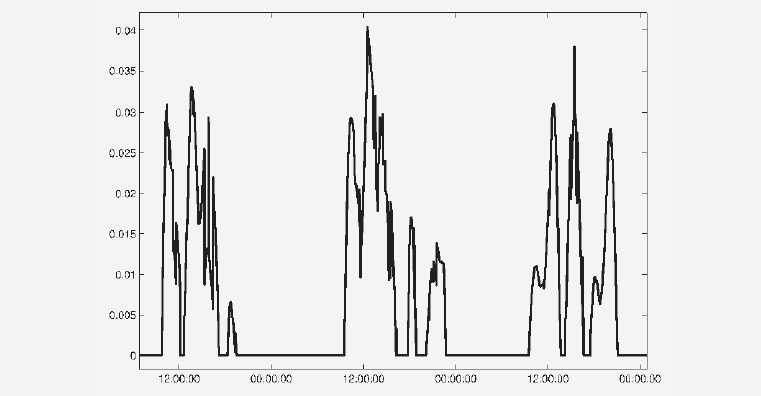
Figure 1
Example of three days of grand average data after daily mean level removal which intends to represent common activities of the facility. Units are normalized and averaged values of the activity epochs and do not associate with the original actigraph data well. Time is given in x-axis
Statistical analysis
To discover associations between functioning and activity behaviours, the Spearman correlation coefficients were calculated between the actigraphy parameters and the ADL score. Spearman correlation analysis was selected because the ADL score is on an ordinal scale. To discover possible u-shape correlations, a regression analysis, including a quadratic term, was performed. We manually selected actigraph data close to the ADL inquiry for the actigraphy parameter extraction.
To study how the functioning level affected the activity behaviours’ indicators (especially the absolute values), we divided the subjects into two groups: whether or not they reported difficulties in performing an activity at most in three daily activities (independent N=22, dependent N=14). The difference in the actigraphy parameters between these two subgroups was studied using the t-test (the Kolmogorov-Smirnov test supported the parameters’ normal distribution).
To study how the parameters behaved on an individual level, we visually observed long-term actigraph recordings and present two cases that we consider to represent well the topic and the monitoring challenge. The analyses were done only qualitatively. We analysed the correlation between weather variables and sleep and activity behaviour in these case recordings. We obtained the daily weather data of the subjects’ residential location during the time periods of the actigraph recordings from the Finnish Metrological Institute (http://en.ilmatieteenlaitos.fi/). The received data contained the daily temperature, atmospheric pressure, wind speed, and sunlight amount (minutes when direct irradiance from the sun was above 120 W/m2). The weather variable data were used as received in the analysis. The analyses were performed in Matlab (Matworks Ltd., www.matworks.com, Natick, Massachusetts, U.S.A.) and in IBM SPSS Statistics (IBM, www.ibm.com, Armonk, U.S.A.).
Results
The Spearman rank correlations between ADL and the actigraphy parameters are presented in Table 1. The ADL questionnaire average score was 27.8 (SD 10.0) for all users, 33.0 (SD 9.2, N=21) for nursing home residents, and 22.2 (SD 8.2, N=15) for assisted living facility residents. The significance levels are adjusted using the Bonferroni method to control for multiple comparisons. The average actigraph data length was 13.7 days and the average missing data was 4.7 percent. Five cases did not have a sleep diary, and the sleep parameters are analysed for 31 cases (SE, TST, AWAKN, and NIGHT ACT). TST (P=0.013 after Bonferroni correction) had a u-shape connection with ADL. The correlations between the activity rhythm parameters and ADL did not change significantly when controlled for activity level (cosinor mesor) and age in a partial Spearman correlation analysis.
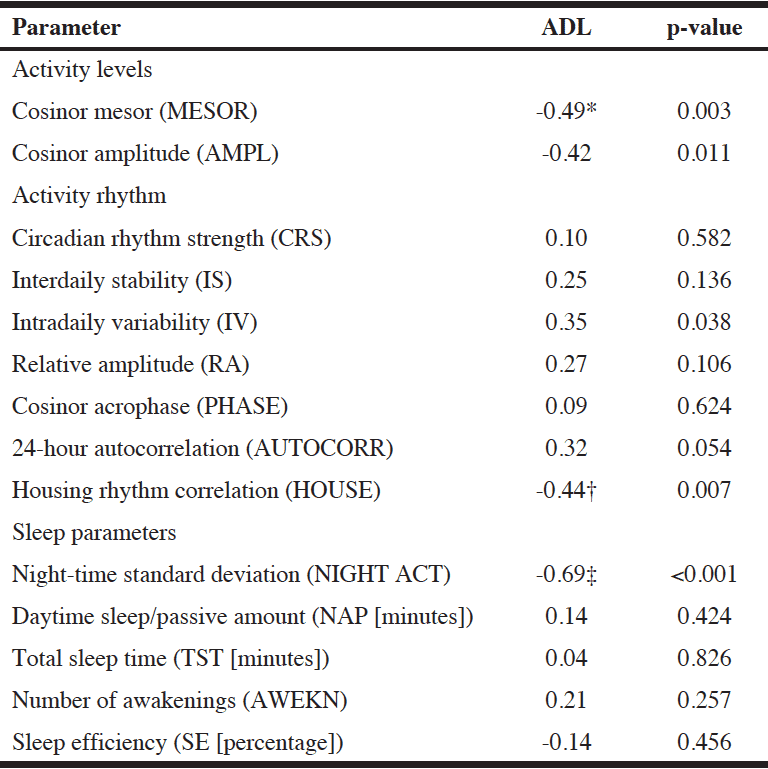
Table 1
Spearman rank correlation coefficient and p-value of the test between Activities of Daily Living questionnaire (ADL) and actigraphy parameters. Significance levels are corrected and p-values values are not corrected using the Bonferroni method
The significance levels are flagged with *P < 0.05, ‡P < 0.01, †P<0.1
Table 2 presents the t-test analysis results between the two groups of subjects, divided according to the number of the self-reported disabilities.
When observing individual cases the associations between actigraph data changes and estimated functioning state varied between individuals. The indicators and the actigraph data were visually studied via two types of figures (see examples from Fig. 2 and Fig. 3). Sub-figure A shows actigraph data (activity level value for each minute) for each 48 hours in the y-axis starting at midnight. The darker the point is in the Sub-figure A, the higher the activity level for that particular minute is. The actigraph data is smoothened by averaging the values over seven days to highlight the behavioural patterns. The graph type in the Sub-figure A is called double plotted actogram and it is utilized widely in the actigraphy research. The Sub-figure B is a collection of bar diagrams for selected actigraphy parameters for the same time frame as the actogram in the Sub-figure A.
Figure 2 is a case example of actigraph data from a 78-year-old male with an ADL total score of forty at the end of the recording (a score of 14 represents the best and 56 the worst). It may be observed from Sub-figure B that timing of the activity becomes earlier (decreasing trend of PHASE) that is visually observable in Sub-figure A as well. The decreasing activity level (AMPL) is well presented in Sub-figures A and B. In addition NIGHT ACT, IS, and AUTOCORR decreased along the suspected declining condition. However, especially NIGHT ACT increased after the last missing data period (beginning of the period of interest marked with a star in Figure 2 A) and AUTOCORR decreased, which are slightly opposite to the group findings when assuming that the subject’s condition is worsening. CRS and IV both react strongly after the last missing data section, around May 2008 (marked with a star). TST does not have strong, systematic changes during the measurement.
Figure 3 presents the second case example (83-year-old female with an ADL score of 15). There were only minor changes in her activity behaviour in the 1069-day-long actigraph recording. According to Sub-figure A (Fig. 3), evening activity decreases, which is visible in advance of PHASE and the lowering of AMPL. IV mildly increases (more fragmented activity behaviour), indicating slightly deteriorating functioning, in accordance with the related research (14, 15).
External influences may also be sporadically observed in the activity patterns of the case examples. For example, we noticed that in the data of Case 2, PHASE tends to follow a six-month rhythm (according to numerical analysis; autocorrelation and Fourier analysis, data not shown) with the zenith in late June and at the turn of the year. In addition, in the data of Case 2, night-time actigraphy deviation (NIGHT ACT) correlated with daily sunlight duration (Spearman rho= 0.21, P < 0.05); the shorter dark time at night during the summer possibly making sleep more restless. A similar trend was not present in the data of Case 1. However, in Case 1 data the outside temperature and daytime activity level (average of activity values between 8 am and 8 pm) had a negative correlation (rho= -0.25, P< 0.05) between the beginning of the monitoring and the first missing data section (marked with a plus sign in Figure 2), suggesting that during the warmer days, the subject was more passive.
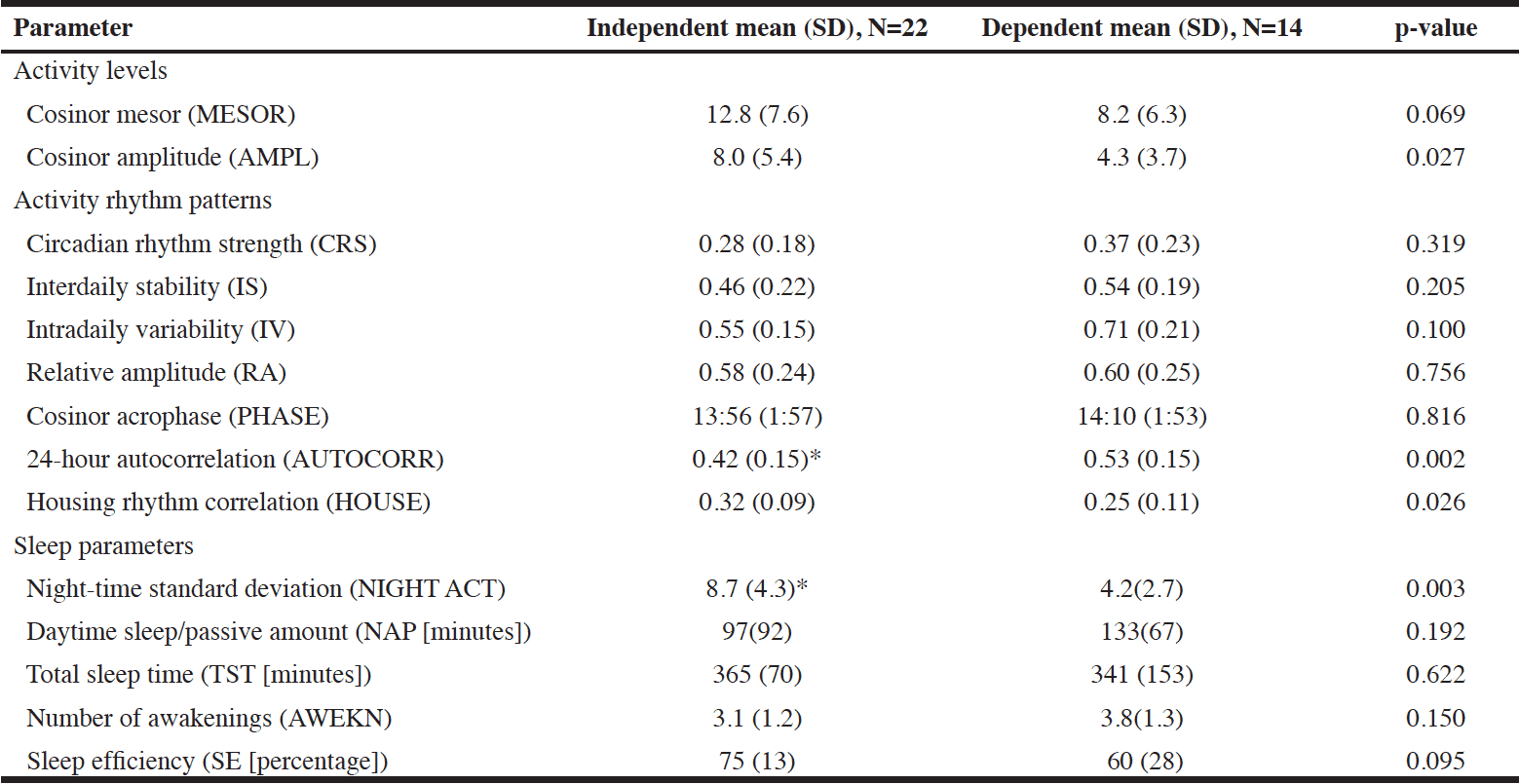
Table 2
Actigraphy parameter values (mean and standard deviation, SD) for two independence groups (the division is based on the number of self-reported disabilities: independent = three or less disabilities). The p-values of the t-test are not Bonferroni corrected
* P<0.05 after Bonferroni correction.
![Figure 2 907-day actigraph recording of Case 1. Sub-figure A is a double plotted actogram for minute-to-minute activity data. Each column presents 48 hours of activity data epochs. The samples are darker if the activity is higher. Pure white coloured sections are missing data. The minute-to-minute activity data are averaged over 7 consecutive days to highlight long-term activity behaviour patterns. Sub-figure B presents selected actigraphy parameters: Cosinor acrophase (PHASE [time]), Circadian rhythm strength (CRS), Intradaily variability (IV), Autocorrelation function of 24-hour lag (AUTOCORR), Interdaily stability (IS), Total sleep time (TST [minutes]), Night-time standard deviation of the actigraphy (NIGHT ACT), and Cosinor amplitude (AMPL [activity level])](https://www.jfrailtyaging.com/wp-content/uploads/2016/06/FIG-4-MERIATHI.gif)
Figure 2
907-day actigraph recording of Case 1. Sub-figure A is a double plotted actogram for minute-to-minute activity data. Each column presents 48 hours of activity data epochs. The samples are darker if the activity is higher. Pure white coloured sections are missing data. The minute-to-minute activity data are averaged over 7 consecutive days to highlight long-term activity behaviour patterns. Sub-figure B presents selected actigraphy parameters: Cosinor acrophase (PHASE [time]), Circadian rhythm strength (CRS), Intradaily variability (IV), Autocorrelation function of 24-hour lag (AUTOCORR), Interdaily stability (IS), Total sleep time (TST [minutes]), Night-time standard deviation of the actigraphy (NIGHT ACT), and Cosinor amplitude (AMPL [activity level])
![Figure 3 A 1069-day actigraph recording named Case 2. Sub-figure A is a double plotted actogram for minute-to-minute activity data. Each column presents 48 hours of activity data epochs. The samples are darker if the activity is higher. Pure white coloured sections are missing data. The minute-to-minute activity data are averaged over 7 consecutive days to highlight long-term activity behaviour patterns. Sub-figure B presents selected actigraphy parameters: Cosinor acrophase (PHASE [time]), Circadian rhythm strength (CRS), Intradaily variability (IV), Autocorrelation function of 24-hour lag (AUTOCORR), Interdaily stability (IS), Total sleep time (TST [minutes]), Night-time standard deviation of the actigraphy (NIGHT ACT), and Cosinor amplitude (AMPL [activity level])](https://www.jfrailtyaging.com/wp-content/uploads/2016/06/FIG-5-MERIATHI.gif)
Figure 3
A 1069-day actigraph recording named Case 2. Sub-figure A is a double plotted actogram for minute-to-minute activity data. Each column presents 48 hours of activity data epochs. The samples are darker if the activity is higher. Pure white coloured sections are missing data. The minute-to-minute activity data are averaged over 7 consecutive days to highlight long-term activity behaviour patterns. Sub-figure B presents selected actigraphy parameters: Cosinor acrophase (PHASE [time]), Circadian rhythm strength (CRS), Intradaily variability (IV), Autocorrelation function of 24-hour lag (AUTOCORR), Interdaily stability (IS), Total sleep time (TST [minutes]), Night-time standard deviation of the actigraphy (NIGHT ACT), and Cosinor amplitude (AMPL [activity level])
Discussion
We studied whether the function status of older adults (the ability to perform daily activities) is associated with activity and sleep behaviours quantified using an actigraph device in free-living, assisted living facilities and nursing home settings. In addition to traditional actigraphy indices, we included methods that intend to quantify subjects’ sleep and activity behaviour responses to external stimuli, such as institute’s daily activities and local weather patterns.
The results indicate an association between the activity level and the ADL score, which is in line with the related literature (25, 28, 29). The finding did not change when controlled for age. However, the activity level did not differ statistically between subjects when divided into two groups, according to the ADL assessment, possibly due to the large variation in the activity levels between the subjects and the moderate number of subjects. The fact that the utilised actigraph was not able to record activity when a subject was out of range of the facility (connection between a receiver unit and a telemetric actigraph unit was lost) might have disturbed the result slightly. In the cosinor analysis the out of range periods were coded as missing data. A more preferred solution in general would be to log the activity data when the sensor is outside the base station range and send the data for the receiver unit afterwards.
Most of the sleep parameters (total sleep time, efficiency, and awakenings) did not show significant correlation with ADL in this dataset. However, according to the polynomial regression (added quadratic term), there tends to be a U-shaped connection between functioning and total sleep time. Similar types of findings with sleep time have been reported in other studies, as well (7, 10). However, there is still little data showing that improving sleep habits would reduce different health risks for older people (30). In addition, it should be considered that the reliability of actigraphy decreases as the fragmentation of sleep increases (5). Night-time activity variability (night-time standard deviation) of the actigraph data had negative correlation with functioning, suggesting that more varying activity during the night could imply better functioning.
Some of the parameters describing diurnal activity patterns indicated association with functioning. The more dependent subjects tend to have a more stable activity rhythm (according to autocorrelation). The similarity of activity rhythm (IS) and the higher activity rhythm association with the facility’s common activities (HOUSE) had a trend with a functioning status (with a significance level of P<0.1). Some of the results actually suggest that, in the institutional settings, varying activity behaviour (according to interdaily stability and night-time variance) might imply better health for people without dementia. This is somewhat opposite than encountered in the related literature for people with dementia (9, 15).
Our indicative results suggest that external stimuli such as facility activities and local weather patterns affect the behaviour of older individuals, and that these influences might differ according to subjects’ levels of functioning. This observation is supported both by quantitative group analysis (housing rhythm correlation with ADL) and qualitative case analysis. In latitudes similar to Finland, the amount of sunlight and the length of the day vary significantly during the year. In addition, the nursing home environment can disrupt stable activity rhythms and sleep/wake patterns through limited exposure to sunlight, long periods spent in bed, physical inactivity, and a disruptive night-time environment (11). These set challenges for people in maintaining their circadian rhythm, and individuals with poor functioning may be more vulnerable to these factors. However, due to the small amount of data, these indications are at the level of a hypothesis and need further study. In addition to more thorough hypothesis testing with a larger sample size, multilevel data analysis would be needed to study how much the different parameters overlap, and how much independent information they actually provide.
The limitation of the study was the small sample size and the heterogeneous subject group, due to which some of the results are indicative rather than conclusive. The telemetric actigraph’s minute-to-minute activity value is also somewhat device specific, and generalising the results to other similar devices should be done with caution. However, the outputs of traditional actigraphs are also different between brands, and it is unlikely that the results would change significantly if a traditional actigraph would be used.
Conclusions
The results suggest that more activity during daytime and more variance in activity patterns are associated with a better functioning status in this study population.
Since the environmental stimuli tend to affect the subject’s activity and rest behaviour, this should be considered in related research. In addition, studying how the subjects react to these environmental stimuli, such as weather patterns or assisted living facility activities, may provide new information on a subject’s functioning state, especially in the preclinical phase. However, more research, especially longitudinal, is needed on the topic. The actigraphy measures describing activity rhythm patterns can potentially be a new insight for monitoring health status, and health changes among older people in the nursing homes and assisted living facilities.
Funding: The analysis was supported by Finnish Cultural Foundation grant 50091953. The data collection was supported by Tekes – the Finnish Funding Agency for Innovation research project “Long term wellness monitoring by wireless low power sensors at real life settings (WSense)”. Vivago Oy supported the research project financially. The sponsors had no role in the design and conduct of the study; in the collection, analysis, and interpretation of data; in the preparation of the manuscript; or in the review or approval of the manuscript.
Acknowledgements: We thank the projects and their personnel, which have made the material possible.
Conflict of interest: Mr Juho Merilahti declares no conflicts of interest. Dr. Korhonen reports grants from TEKES – Finnish Funding Agency for Innovation, grants from Vivago Oy, during the conduct of the study.
References
1. Haveman-Nies A, de Groot LCPGM, van Staveren WA. Dietary quality, lifestyle factors and healthy ageing in europe: The SENECA study. Age Ageing. 2003;324:427-434.
2. WHO. International Classification of Functioning, Disability and Health (ICF). 2015. http://www.who.int/classifications/icf/en/. Accessed 18 April 2016
3. Karnik K, Mazzatti DJ. Review of tools and technologies to assess multi-system functional impairment and frailty. Clinical Medicine: Geriatrics. 2009;20093:1-8.
4. Littner M, Kushida CA, Anderson WM, et al. Practice parameters for the role of actigraphy in the study of sleep and circadian rhythms: An update for 2002. Sleep. 2003;263:337-341.
5. Ancoli-Israel S, Cole R, Alessi C, Chambers M, Moorcroft W, Pollak CP. The role of actigraphy in the study of sleep and circadian rhythms. Sleep. 2003;26:342-392
6. Paavilainen P, Korhonen I, Lötjönen J, et al. Circadian activity rhythm in demented and non-demented nursing-home residents measured by telemetric actigraphy. J Sleep Res. 2005;141:61-68.
7. Goldman SE, Stone KL, Ancoli-Israel S, et al. Poor sleep is associated with poorer physical performance and greater functional limitations in older women. Sleep. 2007;3010:1317-1324.
8. Tranah GJ, Blackwell T, Ancoli-Israel S, et al. Circadian activity rhythms and mortality: The study of osteoporotic fractures. J Am Geriatr Soc. 2010;582:282-291.
9. Carvalho-Bos SS, Riemersma-van Der Lek RF, Waterhouse J, Reilly T, Van Someren EJW. Strong association of the rest-activity rhythm with well-being in demented elderly women. American Journal of Geriatric Psychiatry. 2007;152:92-100.
10. Stone KL, Ancoli-Israel S, Blackwell T, et al. Actigraphy-measured sleep characteristics and risk of falls in older women. Arch Intern Med. 2008;16816:1768-1775.
11. Martin JL, Webber AP, Alam T, Harker JO, Josephson KR, Alessi CA. Daytime sleeping, sleep disturbance, and circadian rhythms in the nursing home. American Journal of Geriatric Psychiatry. 2006;142:121-129.
12. Sharma B, Feinsilver S. Circadian rhythm sleep disorders: An update. Sleep and Biological Rhythms. 2009;72:113-124.
13. Van Someren EJW, Riemersma-Van Der Lek RF. Live to the rhythm, slave to the rhythm. Sleep Medicine Reviews. 2007;116:465-484.
14. Van Someren EJW, Swaab DF, Colenda CC, Cohen W, McCall WV, Rosenquist PB. Bright light therapy: Improved sensitivity to its effects on rest- activity rhythms in alzheimer patients by application of nonparametric methods. Chronobiol Int. 1999;164:505-518.
15. Werth E, Savaskan E, Knoblauch V, et al. Decline in long-term circadian rest-activity cycle organization in a patient with dementia. J Geriatr Psychiatry Neurol. 2002;151:55-59.
16. Sumukadas D, Witham M, Struthers A, McMurdo M. Day length and weather conditions profoundly affect physical activity levels in older functionally impaired people. J Epidemiol Community Health. 2009;634:305-309.
17. Witham MD, Donnan PT, Vadiveloo T, et al. Association of day length and weather conditions with physical activity levels in older community dwelling people. PLoS ONE. 2014;91.
18. Heikkinen E, Waters WE, Brzezinski ZJ. The elderley in eleven countries. A sociomedical survey. Public Health in Europe. 1983;NO. 21:I-XX + 1-231.
19. National TOIMIA network. National TOIMIA network improves the measurement of functioning in Finland. 2011. http://www.thl.fi/toimia/tietokanta. Accessed 18 April 2016.
20. Sarela A, Korhonen I, Lotjonen J, Sola M, Myllymaki M. IST vivago- an intelligent social and remote wellness monitoring system for the elderly. Information Technology Applications in Biomedicine, 2003 4th International IEEE EMBS Special Topic Conference on 2003:362-365.
21. Lötjönen J, Korhonen I, Hirvonen K, Eskelinen S, Myllymäki M, Partinen M. Automatic sleep-wake and nap analysis with a new wrist worn online activity monitoring device vivago WristCare. Sleep. 2003;261:86-90.
22. Van Someren EJW. Improving actigraphic sleep estimates in insomnia and dementia: How many nights? J Sleep Res. 2007;163:269-275.
23. Halberg F, Johnson EA, Nelson W, Runge W, Sothern R. Autorhythmometry -procedures for physiologic self-measurements and their analysis. Physiology Teacher. 1972;1:1-11.
24. Taylor D. Time-series analysis. use of autocorrelation as an analytic strategy for describing pattern and change. West J Nurs Res. 1990;122:254-261.
25. Meadows R, Luff R, Eyers I, Venn S, Cope E, Arber S. An actigraphic study comparing community dwelling poor sleepers with non-demented care home residents. Chronobiol Int. 2010;274:842-854.
26. Lamminmäki E, Saarinen A, Lötjönen J, Partinen M, Korhonen I. Differences in light sleep and deep sleep measured with IST vivago WristCare. 2005;111:1615.
27. Paavilainen P, Korhonen I, Partinen M. Telemetric activity monitoring as an indicator of long-term changes in health and well-being of older people. Gerontechnology. 2005;42.
28. Howell J, Strong BM, Weisenberg J, et al. Maximum daily 6 minutes of activity: An index of functional capacity derived from actigraphy and its application to older adults with heart failure. J Am Geriatr Soc. 2010;585:931-936.
29. Morie M, Reid KF, Miciek R, et al. Habitual physical activity levels are associated with performance in measures of physical function and mobility in older men. J Am Geriatr Soc. 2010;589:1727-1733.
30. Neikrug AB, Ancoli-Israel S. Sleep disorders in the older adult – A mini-review. Gerontology. 2010;562:181-189.