D.M. Konglevoll1, A. Hjartåker1, L.A. Hopstock2, B.H. Strand3,4,5, M. Thoresen6, L.F. Andersen1, M.H. Carlsen1
1. Department of Nutrition, Institute of Basic Medical Sciences, University of Oslo, Oslo, Norway; 2. Department of Community Medicine, UiT The Arctic University of Norway, Tromsø, Norway; 3. Norwegian National Advisory Unit on Ageing and Health, Vestfold Hospital Trust, Tønsberg, Norway; 4. Department of Geriatric Medicine, Oslo University Hospital, Oslo, Norway; 5. Department of Chronic Disease and Ageing, Norwegian Institute of Public Health, Oslo, Norway; 6. Department of Biostatistics, Institute of Basic Medical Sciences, University of Oslo, Oslo, Norway
Corresponding Author: Dina Moxness Konglevoll, University of Oslo, Faculty of Medicine: Universitetet i Oslo Det medisinske fakultet, Oslo, Norway, d.m.konglevoll@medisin.uio.no
J Frailty Aging 2022;11(3)256-266
Published online March 1, 2022, http://dx.doi.org/10.14283/jfa.2022.16
Abstract
Background: Protein intake is suggested as an important dietary factor in the prevention of frailty, however, the influence of lifelong intake remains unclear.
Objectives: The present study investigated the relationship between daily protein intake and patterns of protein intake over 21 years and the risk of pre-frailty/frailty.
Design: Prospective cohort study.
Setting: The population-based Tromsø Study in Tromsø municipality, Norway.
Participants: In total, 1,906 women and 1,820 men aged >45 years in 1994 who participated in both Tromsø4 (1994–95) and Tromsø7 (2015–16).
Measurements: Frailty status in Tromsø7 was measured according to Fried’s phenotype, classifying participants as “robust” (frailty components present: 0), “pre-frail” (1–2) or “frail” (>3). Daily intake of protein was estimated from self-reported habitual dietary intake using food frequency questionnaires and assessed as grams per kilogram bodyweight (g/kg BW) and per megajoule energy intake (g/MJ). The protein–frailty association was assessed via longitudinal and cross-sectional multivariable logistic regression analyses.
Results: The prevalence of pre-frailty and frailty in this study was 27% and 1.0%, respectively. Longitudinal analysis showed that the odds of pre-frailty/frailty decreased by 57% (odds ratio (OR) = 0.43, 95% confidence interval (CI) = 0.31;0.58, p<0.001) with the increase in intake of one additional gram of dietary protein per kg BW. The results obtained from cross-sectional analysis were similar. Tracking analysis showed that, compared to a stable high intake of protein in g/kg BW over time, other patterns of protein intake increased the risk of pre-frailty/frailty. No associations were found between intake of protein in g/MJ and pre-frailty/frailty.
Conclusions: Intake of protein in g/kg BW both in mid-life and later in life was inversely associated with pre-frailty/frailty in older adults. This emphasizes the importance of an adequate protein intake to facilitate healthy ageing in Norwegian older adults.
Key words: Frailty, pre-frailty, protein, nutrition, older adults.
Introduction
A growing ageing population with subsequent age-associated deteriorating health represents one of the most prominent health challenges of the twenty-first century (1). As the proportion of older adults >65 years increases (1), the prevalence of the geriatric syndrome frailty is likely to increase accordingly. Frail individuals experience increased vulnerability to stressors and are at higher risk of negative health outcomes including falls, disability, institutionalization and mortality (2, 3).
Despite the lack of consensus in the definition of frailty, a diversity of scales and indices exists for its operationalization (4). The most widely used definition (4) of physical frailty is the presence of three or more of the five frailty phenotype components proposed by Fried and colleagues in 2001 (3): unintentional weight loss, exhaustion, weakness, slow walking speed and a low physical activity level. The presence of one or two of these criteria indicates pre-frailty, an intermediate stage in which individuals are at high risk of progressing to frailty (5). Owing to the fluctuating nature of the frailty syndrome, these states are also transitional, and therefore, potentially reversible (6).
Poor nutrition is identified as an important modifiable risk factor for frailty, and all components of the frailty phenotype may be influenced directly by this factor (7). Maintenance of a healthy diet is key for preservation of independence during ageing, and in particular a high protein intake has been associated with better physical performance and a lower prevalence of frailty (7-10). Adequate intake of protein contributes to preservation of the muscle protein synthesis and slows down age-associated muscle degeneration and the development of sarcopenia, which facilitates maintenance of muscle mass, physical activity and reduces weight loss (10, 11).
Although several longitudinal (11-15) and cross-sectional (16-18) studies have shown a protective effect of protein consumption on the risk of frailty, other studies have observed no relationship at all (19, 20). The use of study-specific definitions of frailty, and different cut-offs and units to assess protein intake, hampers comparisons among studies (10). Further, most studies on protein intake and risk of frailty have a cross-sectional study design and there are few longitudinal studies with long periods of follow-up (10). Therefore, longitudinal studies are needed to elucidate further the potential role of lifelong protein intake on the risk of frailty.
The aims of the present study were to assess the impact of both previous and current daily intake of protein, as well as tracking patterns of protein intake over 21 years, on the risk of pre-frailty/frailty in Norwegian older adults.
Methods
The Tromsø Study
Data were obtained from the longitudinal population-based Tromsø Study conducted in the municipality of Tromsø, Norway (21). The Tromsø Study consists of seven surveys (Tromsø1–7) carried out between 1974 and 2016, in which full birth cohorts and random samples of the population were invited to participate. In total, 45,473 men and women have participated in one or more of the surveys (participation rate 65%–79%). Data were collected via interviews, questionnaires, physical examinations and biological sampling (21). The present study includes data from Tromsø4 (1994–95) and Tromsø7 (2015–16), the only two study waves in which nutritional intake was estimated.
Study sample
In Tromsø4 (1994–95), all inhabitants aged >25 years (N=37,558) were invited, and 27,158 (72%) participated (22). Invitations were sent by mail accompanied by a short questionnaire, which the participants completed before attendance. At the examination site, participants were given a more comprehensive questionnaire, which included questions about diet, to be completed during the visit or afterwards at home and returned by mail, and they underwent physical examinations including measurement of height in metres (m) and BW in kg in light clothing without shoes (22).
In Tromsø7 (2015–16), all inhabitants >40 years (N=32,591) were invited, and 21,083 (65%) participated (23). On attendance, a sub-sample (n=9,324), were invited to undergo extended examinations (a second visit) approximately two weeks later, in which 8,346 (90%) people attended. This sub-sample consisted of randomly selected participants plus a small extra sample of participants in previous Tromsø studies. For the main examination, participants received invitations by mail with a short printed questionnaire and log-in details to complete this and additional questionnaires online (23). The questionnaires were to be completed before attendance, but technical support was available at the examination site. Participants were subjected to measurements of height and BW, as in Tromsø4, and they received a comprehensive paper-based food frequency questionnaire (FFQ) to be completed during the visit or at home and returned by mail. Participants who attended the second visit underwent comprehensive physical examinations, including measurements of grip strength and walking speed (23).
The present study includes participants from Tromsø4 aged 45–69 years who had participated in Tromsø7 and had data on a minimum of three out of five frailty criteria in Tromsø7. Participants without valid estimated protein intake either in Tromsø4 or in Tromsø7 were excluded, as were participants with energy intakes outside the study-specific cut-offs. In total, 3,726 participants constituted the main analytical sample. For the statistical tests, the sample size was further reduced depending on whether the analyses included estimated protein intake at baseline (n=3,089), follow-up (n=2,507), or at both time points (n=1,908) (Figure 1).
Dashed line marks sub-samples of participants included in statistical analyses
Dietary assessment
Calculations of baseline daily nutrient intake in Tromsø4 were based on self-reported intake of 34 food items from the two study questionnaires provided. Nutrient estimations were performed for those who had answered at least 31 of the 34 questions. Participants with energy intakes outside the <1 (<3,822 kJ/day (914 kilocalories (kcal)/day)) and >99 percentiles (>13,660 kJ/day (3265 kcal/day)) identified from the whole Tromsø4 population were excluded, in accordance with Jacobsen and Nilsen (24). Portion sizes were estimated for each sex on the basis of data from previous dietary surveys in Northern Norway (25, 26). The Norwegian food composition table from 1995 (27) provided the basis for calculations of nutrient intake, supplemented with data from the corresponding Swedish food composition table in the case of missing food composition values (28). A more detailed description of the food and nutrient estimates for Tromsø4 is available in Jacobsen and Nilsen (24).
In Tromsø7, the follow-up nutritional estimates were based on an FFQ developed at the University of Oslo (UiO), designed to collect information on the total diet, including questions on frequency and amount of intake of 261 dietary items (29). Participants who completed less than 90% of the FFQ were excluded, as were participants with extreme energy intakes (<3,948 kJ/day and >21,267 kJ/day (944 kcal/day and 5083 kcal/day)), in accordance with Lundblad et al. (29). Daily intakes of energy and protein were calculated using the food and nutrient calculation system KBS, with database version AE14 at the UiO (KBS, version 7.3.). The food database KBS AE14 is based on the 2014–15 edition of the Norwegian food composition table (http://www.norwegianfoodcomp.no) and supplementary data calculated from recipes and additional databases (30).
Average daily protein intake was expressed in grams (g), grams per megajoule total energy intake (g/MJ), and grams per kilogram bodyweight (g/kg BW). Intake of protein when expressed as g/MJ reflects the proportion of protein in a person’s diet while intake in g/kg BW reflects protein intake relative to BW.
Dietary tracking
For tracking analyses, the participants were allocated to study-specific tertiles of protein intake at baseline and follow-up. Subsequently, the proportion of pre-frail/frail and robust participants with a stable or changed level of protein intake from Tromsø4 to Tromsø7 was identified by cross-tabulation. Stability was presented as the proportion of participants who remained in the same tertile of protein intake between time points, and change was presented as the proportion of participants who decreased or increased their associated tertile of protein intake over time. Tracking coefficients were calculated for each of the two protein variables for pre-frail/frail and robust participants separately using Cohen’s weighted kappa (κw) (31). This is a measure of the level of agreement between tertile memberships at different time points, with membership of the same tertile considered to be perfect agreement and different weighting to movements between adjacent-versus-extreme tertiles (31). Cut-offs proposed by Landis and Koch (32) were used for the interpretation of kappa values. For the logistic regression analyses, a variable of four tracking groups was created: stable low intake (low and medium tertiles), stable high intake (highest tertile), and decreased and increased level of protein intake between Tromsø4 and Tromsø7. The stable high intake was set as the reference category.
Frailty measurement
A modified version of the physical frailty phenotype described by Fried et al. (3) was used to assess frailty in Tromsø7 (Supplementary Table 1). Baseline frailty status was not assessed in Tromsø4 owing to insufficient data.
A low physical activity level was defined as the lowest category, “Mainly reading, watching TV/screen or other sedentary activity”, in the four-level Saltin-Grimby Physical Activity Level Scale (33). Weight loss was defined by the Malnutrition Universal Screening Tool (34) as self-reported involuntary weight loss during the previous 6 months. Exhaustion was defined by a single item from the Hopkins Symptoms Checklist 10 (35): “Have you felt that everything is a struggle during the last week?”, as the two highest categories, “Pretty much” or “Very much”. Low walking speed was defined in accordance with cut-offs for frailty as proposed by Fried et al. (3) using the short physical performance battery test (36). Participants were asked to walk 4 m at their average speed twice, of which the fastest test was recalculated as seconds per 4.752 m (15 feet), and adjusted for sex and height to match the definition of Fried et al. (3). Grip strength (kg) was measured using a Jamar (PLUS+) electric dynamometer. The strongest of three measurements on each hand was recorded as the maximal grip score, as according to the Southampton protocol (37). Low grip strength was defined in accordance with cut-offs for frailty as proposed by Fried et al. (3). For men, low grip strength was defined accordingly for the body mass index (BMI) quartiles; <24, 24.1–26, 26.1–28 and >28 when accompanied by grip strengths (kg) <29, <30, <30, <32, respectively. For women, the corresponding cut-offs were <23, 23.1–26, 26.1–29, >29 and <17, <17.3, <18, <21, respectively. BMI was calculated as measured BW divided by the square of a person’s height (kg/m2).
Participants who fitted none of the above criteria were considered robust, those scoring 1–2 were considered pre-frail, while those with a score >3 were considered frail. Given the low number of participants with frailty score >3 (n=36), the outcome assessed in this study was pre-frail and frail combined (frailty score >1).
Covariates
Baseline (Tromsø4) covariates were selected for descriptive purposes and as potentially confounding factors based on existing literature. Sociodemographic characteristics and lifestyle factors were self-reported by participants in the questionnaires provided.
Smoking status was divided into three groups: never smoked, current daily smoker, and previous daily smoker. Cohabitation was defined based on a combination of the participant’s marital and living status. Participants who were married or living with their spouse/partner, were classified as cohabitants. Level of education was grouped into four categories: 1) primary/(modern) secondary school (7–10 years) , 2) technical/vocational/middle school, 1–2 years senior high school, high school diploma, 3) college/university <4 years and 4) college/university >4 years. The question “Do you feel that you have enough good friends?”, (“yes”/“no”) was included as a measure of the level of social capital and support. Participants were classified as physically active if they reported performing hard physical activity weekly, with sweating or breathlessness, or >3 hours weekly of light activity without sweating or breathlessness. Comorbidity was defined as the self-reported presence of two or more of the following diseases: coronary heart disease (angina pectoris and/or heart attack), stroke, pulmonary disease (asthma and/or chronic bronchitis), peptic ulcer (gastric and/or duodenal ulcer), cancer and diabetes, based on the Charlson Comorbidity Index without weigthing of diseases (38). High alcohol consumption was defined as estimated intake above the upper recommended daily limits set out by the Norwegian Directorate of Health at >10 g for women and >20 g for men (39). The same characteristics were obtained for participants at follow-up (Tromsø7) for sensitivity analyses and descriptive purposes, with the exception of peptic ulcer owing to a lack of information.
Statistical analysis
Differences between pre-frail/frail and robust groups were tested using the Student’s t-test for continuous variables and the chi-square or Fisher’s exact test for categorical variables. Continuous variables are presented as means and standard deviations or 95% confidence intervals (CIs). Categorical variables are presented as counts and proportions.
The association between protein intake and pre-frailty/frailty was examined via multivariable logistic regression analysis in three ways: 1) longitudinal analyses on baseline (Tromsø4) protein intake and 21-year follow-up (Tromsø7) frailty status, 2) cross-sectional analyses on Tromsø7 protein intake and frailty status, and 3) longitudinal analyses on tracking patterns of protein intake between Tromsø4 and Tromsø7, and Tromsø7 frailty status. All effect estimates are presented as odds ratios (OR) with 95% CI.
Primary analyses were solely adjusted for age (Model 1) and subsequently further for baseline (Model 2) and follow-up covariates (Model 4), respectively. The main analytic model, Model 2, was adjusted for age, sex and baseline smoking status, education level, and BMI. Model 4 was adjusted for age, sex and follow-up smoking status, comorbidity and BMI. Multivariable analyses on protein expressed as g/kg BW were not adjusted for BMI. However, to assess the potential influence of energy intake, Models 3 and 5 were additionally adjusted for baseline and follow-up daily energy intake, respectively.
Several supplementary analyses were performed. To assess potential influence of follow-up protein intake, supplementary longitudinal logistic regression analyses were additionally adjusted for Tromsø7 protein intake (Models 6, 7). To account for possible misclassification of participants of robust participants with missing frailty data (n=910), frailty was imputed in individual frailty items in these participants. Imputation was done manually in 25% (n=228), 50% (n=455), 75% (n=683) and 100% (n=910) of cases. Subsequently, Model 2 was run in these four hypothetical study populations. To elucidate further the protein–frailty association, Models 1 and 2 were run on protein intake and frailty score >2, and low grip strength, respectively. Analyses on frailty score >2 excluded participants with frailty score 1 and were run to further account for possible misclassification given that these constituted the vast majority of the pre-frail/frail group. Low grip strength was chosen among the five frailty criteria as a proxy for muscle function.
The multivariable model was built using purposeful selection method with protein intake (g/MJ) as the key exposure variable (40, 41). Following univariate analyses of the covariates previously described, variables with p-values <0.20 or with known clinical relevance (sex) were selected for further inclusion.
Specific diseases were not included in the univariate analysis, only the comorbidity variable as a proxy for disease status. After identification of nonlinear tendencies of the continuous variables age and BMI, these were additionally added to the model in their quadratic forms. No statistically significant interactions were found between biologically plausible variables. All statistical analyses were performed in STATA 16.5. A p-value of <0.05 was considered statistically significant.
Results
Characteristics
The mean age at follow-up was 73 years (Supplementary Table 2). Thirty-six participants (1.0%) were classified as frail and 1,009 (27%) as pre-frail, totalling 1,045 (28%) pre-frail/frail. The prevalence of frailty and pre-frailty increased with age (p<0.001) (Supplementary Table 3).
At baseline, pre-frail/frail participants were slightly older and had higher BW and BMI compared with robust participants, both when all participants were combined, and when stratified by sex (Table 1). A higher proportion of pre-frail/frail than robust participants were daily smokers (37% vs 28%) at baseline, while more robust participants considered their own health as good (77% vs 63%) and were physically active (70% vs 58%). At baseline, pre-frail/frail women were more likely to have the lowest level of education (47% vs 38%). Pre-frail/frail and robust participants did not differ at baseline in terms of cohabitation, self-perceived social support, alcohol consumption or comorbidity, either when men and women were combined or considered separately (Table 1).
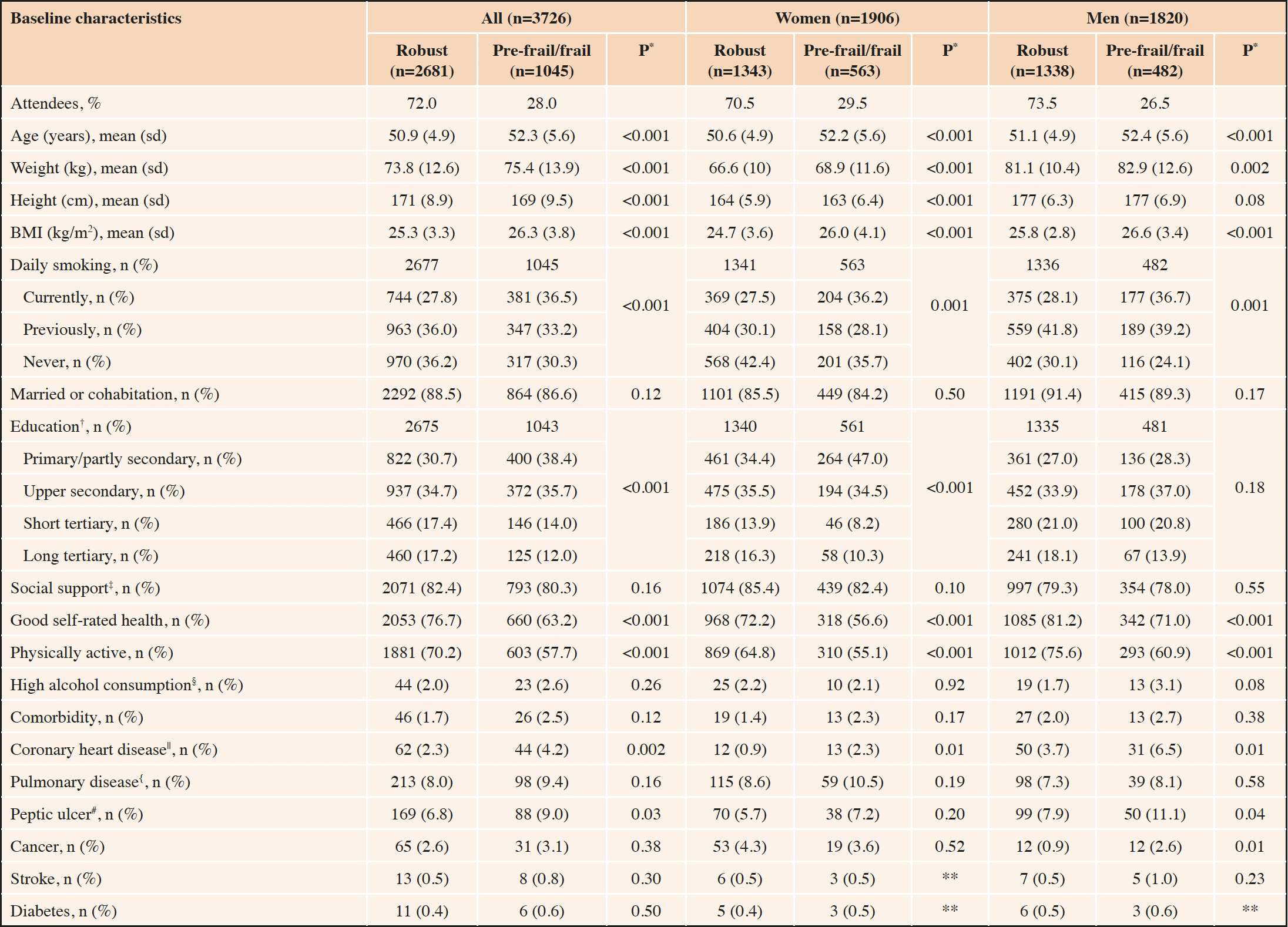
Table 1. Baseline (Tromsø4) characteristics of study participants by Tromsø7 frailty status (n=3726)
BMI: body mass index, sd: standard deviation, MJ: megajoule, BW: bodyweight. N deviates slightly owing to a lack of data on specific variables. *P-value from Student’s t-test for continuous variables and chi-square test for categorical variables between pre-frail/frail and robust women and men. †Primary/secondary school 7–10 years, modern secondary school; technical/vocational/middle school, 1–2 years senior high school or high school diploma (3–4 years); college/university <4 years; college/university >4 years. ‡Self-reported satisfactory number of good friends. §Daily alcohol intake ≥10 g (women) or ≥20 g (men). ||Angina pectoris and/or myocardial infarction. {Asthma and/or chronic bronchitis. #Gastric and/or duodenal ulcer. **No chi-square test performed owing to low n (<5) in cell.
Also at follow-up, pre-frail/frail participants had higher BW and BMI than robust participants (Supplementary Table 2). Compared with robust participants, more pre-frail/frail participants were daily smokers (15% vs 8.0%) and suffered from comorbidity (21% vs 13%), while fewer were satisfied with their own health (45% vs 71%). Pre-frail/frail women were more likely to have completed the lowest level of education (57% vs 43%) and less likely to have high alcohol consumption (17% vs 29%) compared with robust women (Supplementary Table 2).
Tromsø4 participants who did not attend Tromsø7 (n=5,991) were older, had higher BMI, were less physically active (57% vs 65%), had higher prevalence of comorbidity (7.0% vs 2.2%) and slightly higher intake of protein in g/MJ in Tromsø4 compared with those who did (n=4,755) (Supplementary Table 3).
Protein intake
Mean daily protein intake for all participants was 78 g at baseline (Tromsø4) and 93 g at follow-up (Tromsø7) (Table 2). Also, in Tromsø4, mean daily intake was 1.1 g/kg BW and 17 E%, whereas in Tromsø7 the corresponding values were 1.2 g/kg BW and 18 E%, respectively. Both pre-frail/frail and robust participants had higher total and relative daily intake of protein at follow-up (Tromsø7) than at baseline (Tromsø4).
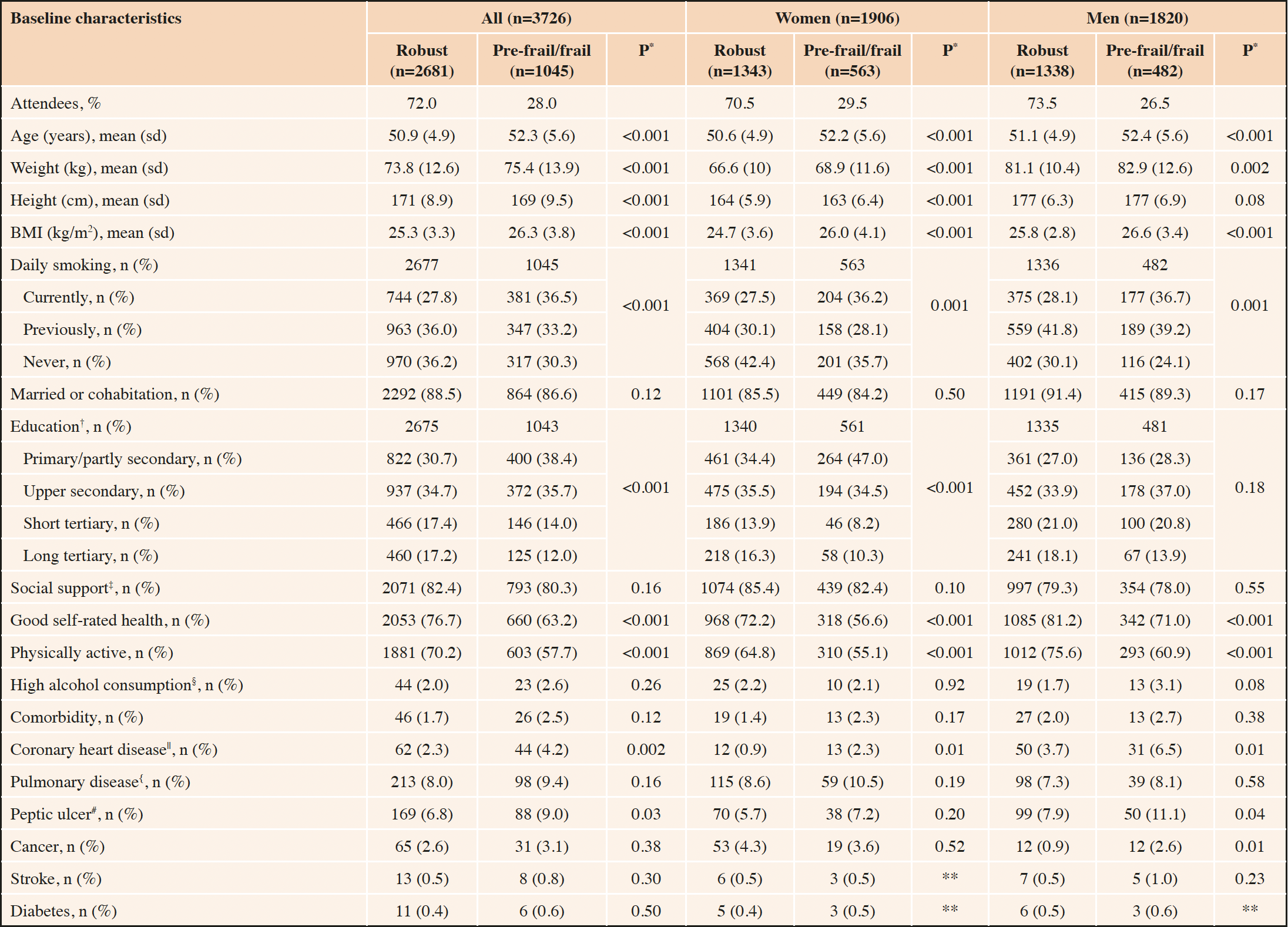
Table 2. Daily nutrient intake in Tromsø4 and Tromsø7 by follow-up (Tromsø7) frailty status (n=3726)
CI: confidence interval, MJ: megajoule, E%: proportion of total energy from protein, BW: bodyweight. N deviates slightly owing to a lack of data on specific variables. Data shown as means and 95% confidence intervals. *P-value from Student’s t-test between daily protein intake and frailty status.
Overall, robust participants had a higher daily intake of protein in g and g/kg BW compared to pre-frail/frail participants at baseline and follow-up (Table 2). In women, robust participants had a higher daily intake of total protein and protein expressed as g/kg BW compared with pre-frail/frail women at baseline (68 g vs 65 g, p=0.003; 1.04 vs 0.97 g/kg BW, p<0.001) and follow-up (90 g vs 84 g, p=0.001; 1.31 vs 1.17 g/kg BW, p<0.001). In men, a marginally higher baseline intake of protein expressed as g/MJ was observed in pre-frail/frail men, compared with robust men (100 vs 99 g/MJ, p=0.02). At follow-up, robust men had slightly higher intake of protein expressed as g/kg BW compared with pre-frail/frail men (1.22 vs 1.17 g/kg BW, p=0.04) (Table 2).
The sub-sample of participants included in the tracking analyses (Figure 1) resembled the main samples with higher observed intakes at follow-up than at baseline (Table 2). Moreover, differences in protein intake between robust and pre-frail/frail groups in the tracking sub-sample were largely similar as described above, except for intake of total protein, which was less likely to differ significantly between groups.
For the tracking of intake of protein expressed as g/kg BW, a trend was observed in which more pre-frail/frail participants had a stable low than high level of intake (53% vs 39%), and a decreased rather than increased (30% vs 27%) tertile of intake (Table 3). In robust participants, a slightly higher proportion had a stable high than low level of intake of protein in g/kg BW (51% vs 49%) between time points. No clear trend was observed for patterns of intake of protein when expressed as g/MJ. Tracking coefficients measured by Cohen’s weighted kappa (0.18–0.25) indicated overall slight to fair tracking of protein intake between time points (Table 3).
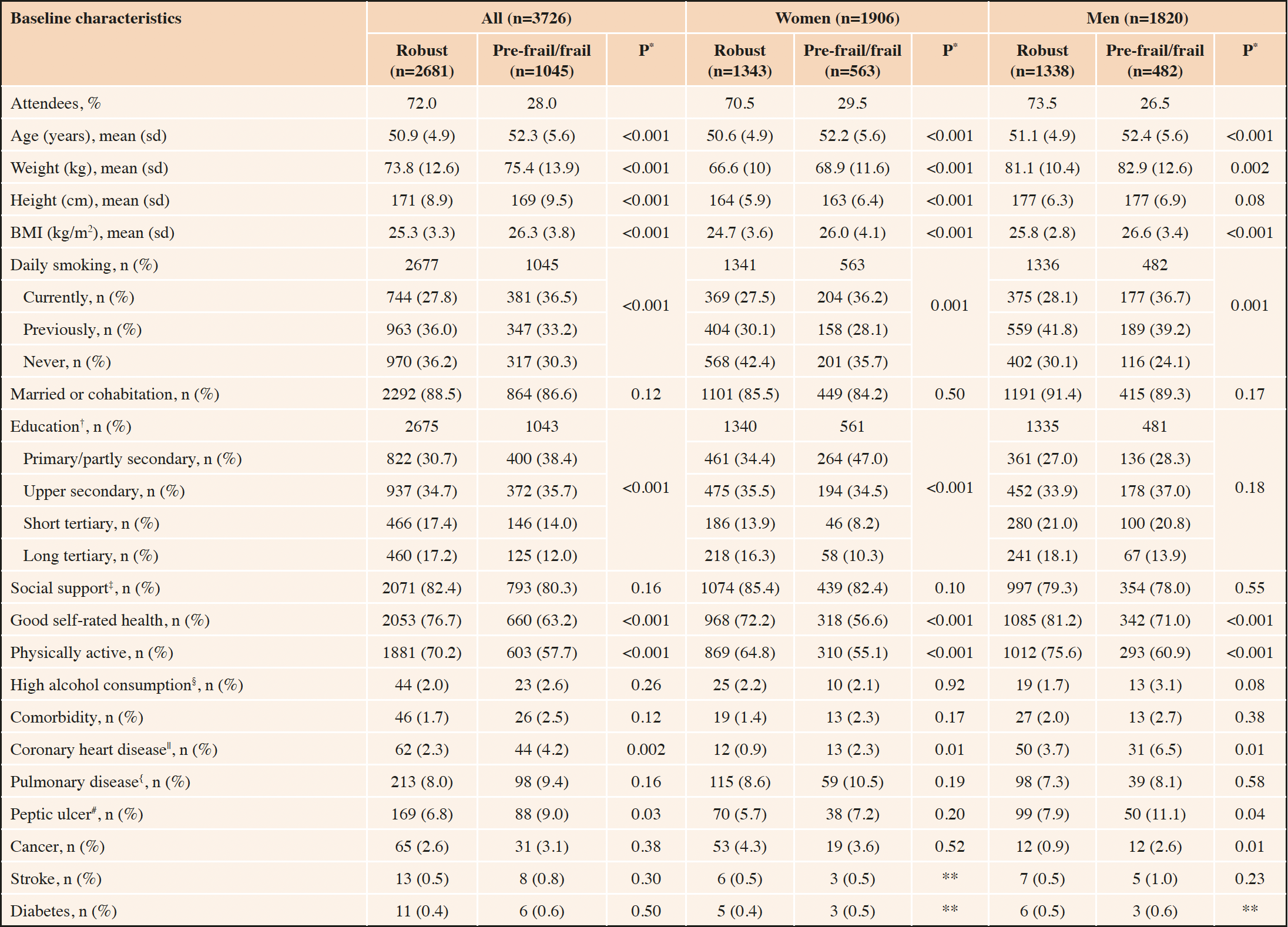
Table 3. Tracking values and proportion of stability of protein intake in pre-frail/frail and robust participants between Tromsø4 and Tromsø7 (n=1908)
MJ: megajoule, BW: bodyweight. nc: no possible change (decrease/increase) in level of intake. *Proportion of participants who changed tertile of protein intake from Tromsø4 to Tromsø7. †Proportion of participants who remained in the same tertile of protein intake from Tromsø4 to Tromsø7. ‡Tracking coefficient of weighted Cohen’s kappa.
Protein intake and risk of pre-frailty/frailty
Longitudinal analyses of protein intake in Tromsø4, expressed as g/kg BW, and pre-frailty/frailty in Tromsø7 showed lower odds of pre-frailty/frailty with increased protein intake both in primary (Model 1) and fully adjusted analyses (Model 2) (OR=0.43, 95%CI=0.31;0.58, p<0.001) (Table 4). Similarly, cross-sectional analyses of protein intake in Tromsø7, expressed as g/kg BW, showed an inverse association with odds of pre-frailty/frailty after adjusting for baseline covariates (Model 2) (OR=0.57, 95%CI=0.46;0.72, p<0.001). All findings remained significant following adjustment for follow-up covariates (Model 4) and/or energy intake (Models 3, 5) (Table 4).
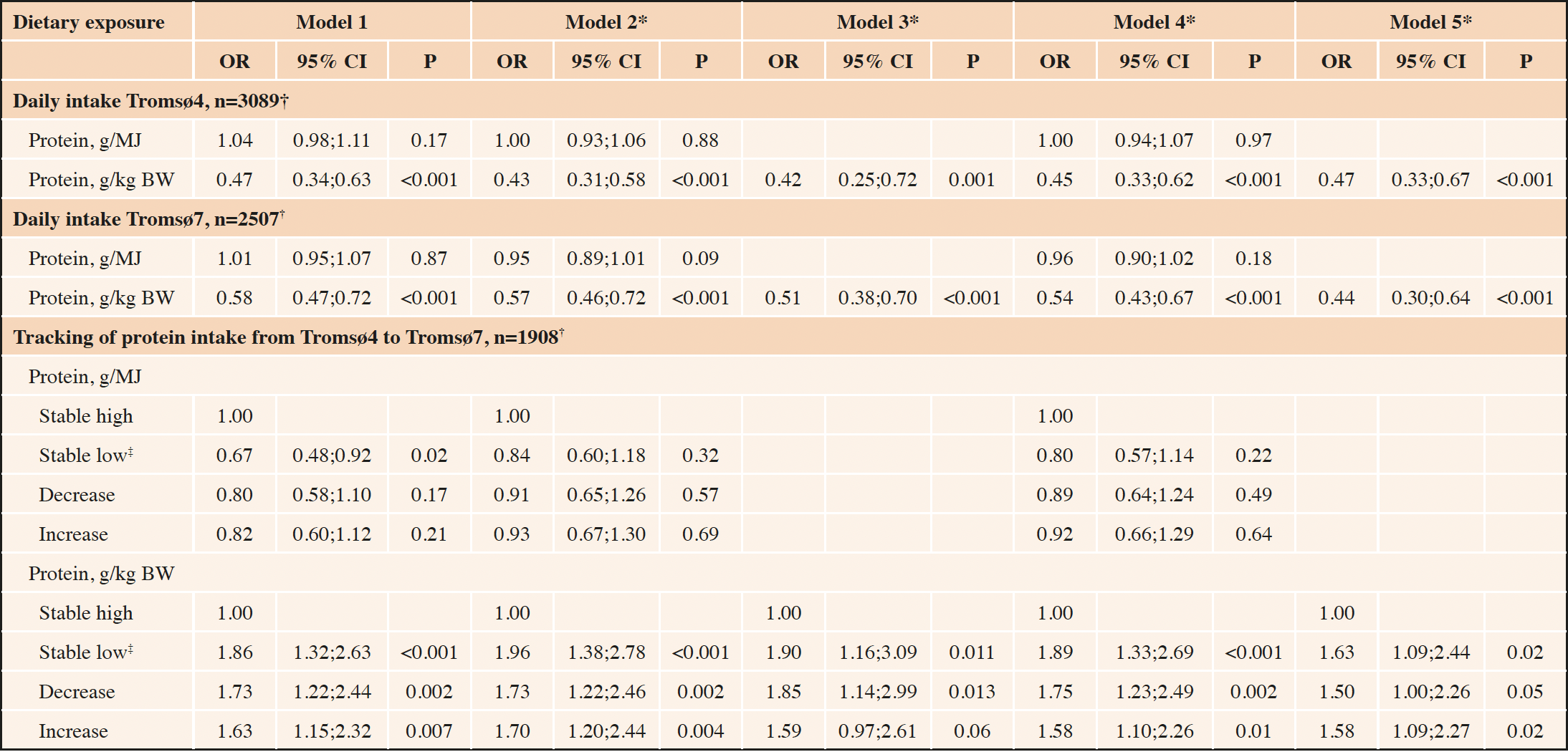
Table 4. Odds ratios (OR) and 95% confidence intervals (CI) for daily intakes of protein in Tromsø4, and Tromsø7, tracking patterns of protein intake from Tromsø4 to Tromsø7, and pre-frailty/frailty in Tromsø7 (n=3726)
MJ: megajoule, BW: bodyweight. OR and 95% CI from logistic regression analyses. *N deviates slightly owing to a lack of data on specific variables. †Analytical sample for Model. ‡Low and medium tertiles. Model 1: Adjusted for baseline age. Model 2: Adjusted for baseline age, sex, smoking, education level and body mass index (not for analyses including protein in g/kg BW). Model 3: Model 2 + additionally adjusted for baseline energy intake (MJ/day). Model 4: Adjusted for Tromsø7 age, sex, smoking, comorbidity and body mass index (not for analyses with protein per g/kg BW). Model 5: Model 4 + additionally adjusted for Tromsø7 energy intake (MJ/day)
Results from tracking analyses of protein intake, expressed as g/kg BW, showed that participants with a stable low intake or who changed their tertile of protein intake between time points had higher odds of pre-frailty/frailty than those with a stable high level of intake. Specifically, a stable low protein intake (OR=1.96, 95%CI=1.38;2.78, p<0.001), a decreased (OR=1.73, 95%CI=1.22;2.46, p=0.002) or an increased tertile of protein intake over time (OR=1.70, 95%CI=1.20;2.44, p=0.004) increased the risk of pre-frailty/frailty in Tromsø7 (Model 2, Table 4). Following additional adjustment for energy intakes, the patterns increased and decreased level of protein intake (in g/kg BW) were not significantly (Model 3) and borderline significantly (Model 5) associated with pre-frailty/frailty in Tromsø7 (Table 4).
For intake of protein in g/MJ, age-adjusted tracking analysis (Model 1) showed that participants with a stable low intake over time had lower odds of pre-frailty/frailty in Tromsø7 (OR=0.67, 95%CI=0.48;0.92, p=0.02) than participants with a stable high intake, although this was no longer significant after further adjustments (Table 4). No other associations were found between intake of protein in g/MJ and pre-frailty/frailty in Tromsø7.
Supplementary analyses with additional adjustment for protein intake in Tromsø7 supported the findings of an inverse association between intake of protein in g/kg BW and risk of pre-frailty/frailty (Supplementary Table 5). Similarly, sensitivity analyses with imputed frailty data showed lower odds of pre-frailty/frailty with increased daily intake of protein in g/kg BW, at all levels of imputation. Also, with imputations, the only patterns of protein intake associated with increased risk of pre-frailty/frailty were a stable low, and a decreased level of intake (Supplementary Table 6). Results were similar for sensitivity analyses on daily intake of protein in g/kg BW and frailty score >2. A stable low level of intake was associated with increased risk of frailty score >2 (Supplementary Table 7). For low grip strength, cross-sectional analyses showed an inverse association with daily intake of protein in g/kg BW. Tracking analyses showed higher odds of low grip strength in Tromsø7 in participants with decreased level of protein intake over time (Supplementary Table 8).
Discussion
Daily intake of protein expressed as g/kg BW in adulthood and older age was inversely associated with risk of pre-frailty/frailty in older age. Tracking analysis showed that, compared to a stable high intake of protein in g/kg BW over time, different patterns of protein intake increased the risk of pre-frailty/frailty. No significant associations were found between intake of protein relative to energy (in g/MJ) and pre-frailty/frailty.
In line with the observed beneficial effects of increased intake of protein expressed as g/kg BW on risk of pre-frailty/frailty as seen from longitudinal analyses, were findings reported by Beasley et al. in the Women’s Health Initiative cohort (11). Beasley and colleagues found that a 20% increased intake of protein (g/kg BW) calibrated by 24-hour urinary nitrogen was associated with a 35% lower risk of frailty and a 22% lower risk of pre-frailty among 24,417 older women over a 3-year follow-up (11). In support of this, The Newcastle 85+ study showed that increased intake of protein in g/kg BW adjusted to normal BMI for older adults (22–27 kg/m2) decreased the likelihood of transitioning from pre-frail to frail over five years in the oldest individuals (>85 years) (15). Further, an American 10-year longitudinal study observed fewer health problems, including a study-specific definition of frailty, amongst community-dwelling women over 60 years with a daily protein intake >1.2 g/kg BW compared with those consuming <0.8 g/kg BW (14). The lower cut-off at 0.8 g/kg BW was set according to the current Recommended Daily Allowance for protein intended for healthy adults and older adults (42), and the upper cut-off (>1.2 g/kg BW) was set as emerging evidence has suggested that older adults need a higher protein intake to maintain muscle mass and function (14, 39, 43, 44). However, using these cut-offs (<0.8 and >1.2 g/kg BW), a prospective cohort study of Dutch adults (>45 years) did not observe any association between protein intake and risk of frailty (19).
In line with our findings from cross-sectional analyses on intake of protein relative to BW, Rahi and colleagues (16) found that, in older French community-dwellers, daily protein intake >1 g/kg BW was significantly associated with a lower prevalence of frailty when compared with those consuming less protein. Conversely, Bollwein et al. (45) found no association between quartiles of protein intake (g/kg BW) and risk of frailty. Of note, the cross-sectional studies suffer the risk of reverse causality (46). The findings from supplementary analyses performed with imputed frailty data and on frailty score >2 and low grip strength emphasize the protective effect of consuming sufficient amounts of protein relative to one’s BW.
The lack of association between intake of protein in g/MJ and risk of pre-frailty/frailty in this study, was somewhat confusing. However, the null findings observed in the longitudinal analyses are in agreement with Shikany et al. (20), who did not observe any association between quartiles of protein E% and frailty amongst older US men over a 4.6-year follow-up period. They did, however, show an inverse association between overall diet quality and risk of frailty. Furthermore, a Japanese prospective cohort found higher total protein intake to be negatively associated with pre-frailty/frailty development in older adults over 2 years; however, the results were no longer statistically significant after additional adjustment for energy intake. The authors suggested this indicated that increased energy intake mediated the contributions of protein intake towards reducing frailty development (47). This hypothesis was tested in our study by performing risk analyses stratified by quartiles of energy intake, however, this did not influence the results notably (data not shown). Moreover, considering the observed higher BW and BMI of pre-frail/frail participants, it could be speculated that the observed increased risk of pre-frailty/frailty from intake of protein in g/kg BW is in fact due to differences in BW and body composition between groups, however, this was not investigated further due to lack of body composition data.
Contradictory to our findings, other studies have observed a relationship between energy-adjusted protein intake and frailty. Sandoval-Insausti and colleagues (12) found an inverse association between quartiles of total protein intake adjusted for energy and risk of frailty over 3.5 years amongst Spanish community-dwellers (>60 years). The aforementioned findings of Beasley et al. of reduced risk of frailty with higher protein intake in g/kg BW persisted when calibrated protein intake was expressed as E% (11). In addition, two cross-sectional studies performed among community-dwelling older Italians (18) and Japanese women (17), respectively, found an inverse association between quintiles of daily intake of energy-adjusted protein and frailty.
Tracking of protein intake and risk of frailty
The low tracking values obtained in the present study are comparable with other tracking studies on lifestyle variables, and their magnitude is impaired by the variables’ moderate reproducibility and the long follow-up period (48-50). For intake of protein expressed as g/kg BW, the observed opposing trends of patterns of protein intake between pre-frail/frail versus robust participants were not clearly reflected in the results, as all patterns of protein intake over time, except for a stable high intake, was associated with an increased risk of pre-frailty/frailty. However, the results from the sensitivity analyses indicate that a low or decreased level of intake is more crucial in terms of frailty risk than any pattern of increased protein intake. Most notably, tracking analyses on frailty score >2 and low grip strength should be interpreted with caution given the high level of uncertainty observed in the risk estimates.
Strengths and limitations
Major strengths of the current study are the prospective design, which allowed for follow-up of a large population-based sample over two decades, and the use of validated instruments to measure frailty components. Additionally, the assessment of the protein–frailty association in both longitudinal and cross-sectional analyses provides a more thorough understanding of the relationship than results from just one or the other.
A key limitation is that this study suffers from selective drop-out of participants with overall poorer health. This attrition contributes to the existing risk of selection bias associated with population-based studies, given that study participants tend to have both better health and higher socioeconomic status than non-participants (51). Non-attendance of the frailest individuals invited to Tromsø7 may have influenced the observed associations and contributed to the low observed prevalence of frailty. The observed prevalence was lower than reported in community dwellers worldwide (52), in Europe (53), and amongst participants >70 years in Tromsø5 in 2001 (54). In addition, missing frailty data might have contributed further to the low frailty prevalence. However, results from sensitivity analyses in participants with imputed frailty data, supported the main findings of an inverse association between intake of protein in g/kg BW and risk of pre-frailty/frailty.
Aside from the problem of selection bias, the relatively good health of the study participants may also reflect research showing that today’s older adults are notably stronger than previous generations (55). Therefore, one could argue that Fried’s cut-offs (3) are not optimal for identifying frailty accurately in the present study population, considering that these cut-offs are population-specific to older Americans in 2001.
This study suffers from the risk of misclassification given the combination of participants originally classified as pre-frail or frail in the more heterogeneous group ‘pre-frail/frail’ group and because the majority of participants in this group had a frailty score of 1. Consequently, there is a risk that practically healthy participants were grouped together with the genuinely frail. This was addressed to some extent in the results of the sensitivity analyses on frailty score >2, which for the most part supported the main results. Nonetheless, the majority of the pre-frail/frail participants were in fact pre-frail, and therefore comparisons with studies on frail participants are weakened.
Another important limitation is the risk of information bias introduced by self-reported variables, including the frailty criteria physical activity level, exhaustion and weight loss, and the dietary exposure variables and adjustment covariates. At both time points, the observed relative protein intake of the participants was in line with current Norwegian dietary recommendations for both healthy adults (0.8–1.5 g/kg BW, 10–20 E%) and older adults (1.1–1.3 g/kg BW, 15–20 E%) (39). However, the comparability of the protein estimates between the two surveys was reduced substantially because they were based on distinctly different questionnaires and dietary information, and different food composition databases were used for the protein calculations. The estimated nutritional intake in Tromsø7 was based on a much higher number of dietary items than in Tromsø4, and it is natural to assume that the reported intake will increase with increased number of foods asked about. Additionally, portion sizes in Tromsø4 were estimated on the basis of previous dietary surveys whereas they were specifically asked for in the Tromsø7 FFQ. Therefore, there is a risk that the observed increased daily intake of protein over time may be attributable to methodological differences.
The two relative protein variables measured in this study have different sources of error according to their respective adjustment variables, given that BW was measured objectively whilst estimated energy intake was based on self-reported data. Moreover, the protein variables reflect the participants’ protein intake in two different ways. By adjusting for energy, one can to a certain extent reduce the confounding effect of energy in the analyses, and account for the influence of other factors that affect energy intake, such as physical activity level, body composition, and metabolism (56). On the other hand, changes in BW may themselves influence protein intake in g/kg BW. Therefore, observed changes in intake of protein in g/kg BW may be explained either by changes in protein intake, BW or both.
Unfortunately, there is no validation study on the nutritional data obtained from Tromsø4, but the estimated proportions of energy obtained from macronutrients were comparable to data in the first two Norkost surveys (1994–95, 1997), intended to be representative of the Norwegian population aged 16–79 years (24, 57). The much more comprehensive FFQ used in Tromsø7 has been validated (58-60) and is considered a suitable tool for dietary assessment in large population surveys.
In addition to being self-reported, the majority of the study covariates were dichotomized which led to loss of information and potential for residual confounding. The findings from the present study are generalizable to community-dwelling Norwegian adults and older adults, as long as these limitations are kept in mind. Specifically, the generalizability of the results from the tracking analyses is impaired by the use of study-specific measures as opposed to objective cut-offs for protein intake (50).
One further limitation of the study is that there are no repeated measurements of frailty. However, no information on frailty status was available at baseline. Similarly, repeated measurements of protein intake between Tromsø4 and Tromsø7 might have added to the study but no such data were available. There were also no data available to assess the influence of different sources of protein (plant versus animal), the amount of protein intake per meal, and the timing of protein intake.
In conclusion, the vast majority of the pre-frail/frail participants in this population-based study were pre-frail. The results highlight the significant associations between protein intake, BW and frailty development, particularly via the transitional state of pre-frailty. These findings emphasize the importance of consuming an adequate amount of protein in adulthood and of complying with current dietary recommendations in order to prevent age-related loss of muscle mass and function.
Funding: This work was funded by the Institute of Basic Medical Sciences, University of Oslo with supplementary funds from Aktieselskabed Freia Chocolade Fabriks Medisinske fond for 2019 (8052) and the Throne Holst Nutrition Research Foundation (UNIFOR). The sponsors had no role in the design and conduct of the study; in the collection, analysis, and interpretation of data; in the preparation of the manuscript or in the review or approval of the manuscript.
Acknowledgements: We thank Bjarne K. Jacobsen and Tom Wilsgaard for their help with handling specific variables from the Tromsø Study.
Conflict of interest (disclosure statements): All authors declare no conflict of interest. The funding sources had no role in the design and conduct of the study.
Ethical standards: The study was conducted in accordance with the Declaration of Helsinki, and the project was approved by Regional Committees for Medical and Health Research Ethics (REK; 2019/43798). Informed consent was obtained from all participants for being included in the study.
Open Access: This article is distributed under the terms of the Creative Commons Attribution 4.0 International License (http://creativecommons.org/licenses/by/4.0/), which permits use, duplication, adaptation, distribution and reproduction in any medium or format, as long as you give appropriate credit to the original author(s) and the source, provide a link to the Creative Commons license and indicate if changes were made.
References
1. United Nations Department of Economic and Social Affairs PD. World Population Ageing 2020 Highlights: Living arrangements of older persons. New York, ST/ESA/SER.A/451; 2020.
2. Chen X, Mao G, Leng SX. Frailty syndrome: an overview. Clin Interv Aging. 2014;9:433-41. https://doi.org/10.2147/CIA.S45300
3. Fried LP, Tangen CM, Walston J, Newman AB, Hirsch C, Gottdiener J, et al. Frailty in Older Adults: Evidence for a Phenotype. J Gerontol A Biol Sci Med Sci. 2001;56(3):M146-M57. https://doi.org/10.1093/gerona/56.3.m146
4. Buta BJ, Walston JD, Godino JG, Park M, Kalyani RR, Xue Q-L, et al. Frailty assessment instruments: Systematic characterization of the uses and contexts of highly-cited instruments. Ageing Res Rev. 2016;26:53-61. https://doi.org/10.1016/j.arr.2015.12.003
5. Xue Q-L. The Frailty Syndrome: Definition and Natural History. Clin Ger Med. 2011;27(1):1-15. https://doi.org/10.1016/j.cger.2010.08.009
6. Kojima G, Taniguchi Y, Iliffe S, Jivraj S, Walters K. Transitions between frailty states among community-dwelling older people: A systematic review and meta-analysis. Ageing Res Rev. 2019;50:81-8. https://doi.org/10.1016/j.arr.2019.01.010
7. Bonnefoy M, Berrut G, Lesourd B, Ferry M, Gilbert T, Guerin O, et al. Frailty and nutrition: searching for evidence. J Nutr Health Aging. 2015;19(3):250-7. https://doi.org/10.1007/s12603-014-0568-3
8. Lorenzo-López L, Maseda A, de Labra C, Regueiro-Folgueira L, Rodríguez-Villamil JL, Millán-Calenti JC. Nutritional determinants of frailty in older adults: A systematic review. BMC Geriatrics. 2017;17(1):108. https://doi.org/10.1186/s12877-017-0496-2
9. Yannakoulia M, Ntanasi E, Anastasiou CA, Scarmeas N. Frailty and nutrition: From epidemiological and clinical evidence to potential mechanisms. Metabolism. 2017;68:64-76. https://doi.org/10.1016/j.metabol.2016.12.005
10. Coelho-Junior HJ, Rodrigues B, Uchida M, Marzetti E. Low Protein Intake Is Associated with Frailty in Older Adults: A Systematic Review and Meta-Analysis of Observational Studies. Nutrients. 2018;10(9):14. https://doi.org/10.3390/nu10091334
11. Beasley JM, LaCroix AZ, Neuhouser ML, Huang Y, Tinker L, Woods N, et al. Protein Intake and Incident Frailty in the Women’s Health Initiative Observational Study. J Am Geriatr Soc. 2010;58(6):1063-71. https://doi.org/10.1111/j.1532-5415.2010.02866.x
12. Sandoval-Insausti H, Pérez-Tasigchana RF, López-García E, García-Esquinas E, Rodríguez-Artalejo F, Guallar-Castillón P. Macronutrients Intake and Incident Frailty in Older Adults: A Prospective Cohort Study. J Gerontol A Biol Sci Med Sci. 2016;71(10):1329-34. https://doi.org/10.1093/gerona/glw033
13. Houston DK, Nicklas BJ, Ding J, Harris TB, Tylavsky FA, Newman AB, et al. Dietary protein intake is associated with lean mass change in older, community-dwelling adults: the Health, Aging, and Body Composition (Health ABC) Study. Am J Clin Nutr. 2008;87(1):150-5. https://doi.org/10.1093/ajcn/87.1.150
14. Vellas BJ, Hunt WC, Romero LJ, Koehler KM, Baumgartner RN, Garry PJ. Changes in Nutritional Status and Patterns of Morbidity Among Free-Living Elderly Persons: A 10-Year Longitudinal Study. Nutrition. 1997;13(6):515-9. https://doi.org/10.1016/s0899-9007(97)00029-4
15. Mendonça N, Kingston A, Granic A, Jagger C. Protein intake and transitions between frailty states and to death in very old adults: the Newcastle 85+ study. Age and Ageing. 2019;49(1):32-8. https://doi.org/10.1093/ageing/afz142
16. Rahi B, Colombet Z, Gonzalez-Colaço Harmand M, Dartigues J-F, Boirie Y, Letenneur L, et al. Higher Protein but Not Energy Intake Is Associated With a Lower Prevalence of Frailty Among Community-Dwelling Older Adults in the French Three-City Cohort. J Am Med Dir Assoc. 2016;17(7):672.e7-.e11. https://doi.org/10.1016/j.jamda.2016.05.005
17. Kobayashi S, Asakura K, Suga H, Sasaki S. High protein intake is associated with low prevalence of frailty among old Japanese women: a multicenter cross-sectional study. Nutr J. 2013;12:164. https://doi.org/10.1186/1475-2891-12-164
18. Bartali B, Frongillo EA, Bandinelli S, Lauretani F, Semba RD, Fried LP, et al. Low Nutrient Intake Is an Essential Component of Frailty in Older Persons. J Gerontol A Biol Sci Med Sci. 2006;61(6):589-93. https://doi.org/10.1093/gerona/61.6.589
19. Schoufour JD, Franco OH, Kiefte-de Jong JC, Trajanoska K, Stricker B, Brusselle G, et al. The association between dietary protein intake, energy intake and physical frailty: results from the Rotterdam Study. Br J Nutr. 2019;121(4):393-401. https://doi.org/10.1017/s0007114518003367
20. Shikany JM, Barrett-Connor E, Ensrud KE, Cawthon PM, Lewis CE, Dam TT, et al. Macronutrients, Diet Quality, and Frailty in Older Men. J Gerontol A Biol Sci Med Sci. 2014;69(6):695-701. https://doi.org/10.1093/gerona/glt196
21. UiT. The Arctic University of Norway. Tromsøunderøskelsen: Spørsmål og svar [Internet]. 2020 [cited 2021 March 11]. Available from: https://uit.no/research/tromsoundersokelsen/project?pid=706805.
22. UiT. The Arctic University of Norway. The Fourth Tromsø Study [Internet]. 2020 [cited 2021 Jan 04]. Available from: https://uit.no/research/tromsostudy/project?pid=708901.
23. UiT. The Arctic University of Norway. The sevent survey of the Tromsø Study [Internet]. 2020 [cited 2021 Jan 04]. Available from: https://uit.no/research/tromsostudy/project?pid=708909.
24. Jacobsen BK, Nilsen H. High education is associated with low fat and high fibre, beta-carotene and vitamin C – Computation of nutrient intake based on a short food frequency questionnaire in 17,265 men and women in the Tromsø Study. Norsk Epidemiologi. 2000;10(1):57-62. https://doi.org/10.5324/nje.v10i1.515
25. Nilsen H, Utsi E, Bønaa K. Dietary and nutrient intake of a Sami population living in traditional reindeer herding areas in north Norway: comparisons with a group of Norwegians. Int J Circumpolar Health. 1999;58(2):120-33.
26. Grimsgaard S, Bønaa KH, Hansen J-B, Nordøy A. Highly purified eicosapentaenoic acid and docosahexaenoic acid in humans have similar triacylglycerol-lowering effects but divergent effects on serum fatty acids. Am J Clin Nutr. 1997;66(3):649-59. https://doi.org/10.1093/ajcn/66.3.649
27. Rimestad AH, Statens ernæringsråd, Statens næringsmiddeltilsyn. Den Store matvaretabellen 1995. Oslo: Universitetsforlaget; 1995.
28. Statens Livsmedelsverk. Livsmedelstabell: Energi och näringsämnen (nutrition tables). Uppsala, Nordstedts tryckeri. 1993.
29. Lundblad MW, Andersen LF, Jacobsen BK, Carlsen MH, Hjartåker A, Grimsgaard S, et al. Energy and nutrient intakes in relation to National Nutrition Recommendations in a Norwegian population-based sample: the Tromsø Study 2015–16. Food Nutr Res. 2019;63. https://dx.doi.org/10.29219%2Ffnr.v63.3616
30. Norberg N, Hjartåker A, Carlsen MH. Fettsyreverdier er nå tilgjengelig i forskningsverktøyet KBS. Norsk tidsskrift for ernæring. 2018;1:19-22.
31. Cohen J. Weighted kappa: Nominal scale agreement provision for scaled disagreement or partial credit. Psychol Bull. 1968;70(4):213-20. https://psycnet.apa.org/doi/10.1037/h0026256
32. Landis JR, Koch GG. The Measurement of Observer Agreement for Categorical Data. Biometrics. 1977;33(1):159-74. https://doi.org/10.2307/2529310
33. Grimby G, Börjesson M, Jonsdottir IH, Schnohr P, Thelle DS, Saltin B. The «Saltin-Grimby Physical Activity Level Scale» and its application to health research. Scand J Med Sci Sports. 2015;25 Suppl 4:119-25. https://doi.org/10.1111/sms.12611
34. Malnutrition Advisory Group (MAG). MUST Nutritional Screening. Redditch, Worcestershire: BAPEN; 2008.
35. Strand BH, Dalgard OS, Tambs K, Rognerud M. Measuring the mental health status of the Norwegian population: a comparison of the instruments SCL-25, SCL-10, SCL-5 and MHI-5 (SF-36). Nord J Psychiatry. 2003;57(2):113-8. https://doi.org/10.1080/08039480310000932
36. Guralnik JM, Simonsick EM, Ferrucci L, Glynn RJ, Berkman LF, Blazer DG, et al. A Short Physical Performance Battery Assessing Lower Extremity Function: Association With Self-Reported Disability and Prediction of Mortality and Nursing Home Admission. J Gerontol. 1994;49(2):M85-M94. https://doi.org/10.1093/geronj/49.2.M85
37. NIHR Southampton BRC. Procedure for Measuring Han Grip Strength Using the JAMAR Dynamometer. Loughborough, UK: NHS, NIHR; 2014.
38. Charlson ME, Pompei P, Ales KL, MacKenzie CR. A new method of classifying prognostic comorbidity in longitudinal studies: Development and validation. J Chronic Dis. 1987;40(5):373-83. https://doi.org/10.1016/0021-9681(87)90171-8
39. Nordic Council of Ministers. Nordic nutrition recommendations 2012 : integrating nutrition and physical activity. 5th ed. Copenhagen: Nordic Council of Ministers; 2014.
40. Zhang Z. Model building strategy for logistic regression: purposeful selection. Ann Transl Med. 2016;4(6):111-7. https://dx.doi.org/10.21037%2Fatm.2016.02.15
41. Hosmer DW, Lemeshow S, Sturdivant RX. Applied logistic regression. 3rd ed. Hoboken, N.J.: John Wiley & Sons; 2013.
42. Rand WM, Pellett PL, Young VR. Meta-analysis of nitrogen balance studies for estimating protein requirements in healthy adults. Am J Clin Nutr. 2003;77(1):109-27. https://doi.org/10.1093/ajcn/77.1.109
43. Bauer J, Biolo G, Cederholm T, Cesari M, Cruz-Jentoft AJ, Morley JE, et al. Evidence-Based Recommendations for Optimal Dietary Protein Intake in Older People: A Position Paper From the PROT-AGE Study Group. J Am Med Dir Assoc. 2013;14(8):542-59. https://doi.org/10.1016/j.jamda.2013.05.021
44. Deutz NEP, Bauer JM, Barazzoni R, Biolo G, Boirie Y, Bosy-Westphal A, et al. Protein intake and exercise for optimal muscle function with aging: Recommendations from the ESPEN Expert Group. Clin Nutr. 2014;33(6):929-36. https://doi.org/10.1016/j.clnu.2014.04.007
45. Bollwein J, Diekmann R, Kaiser MJ, Bauer JM, Uter W, Sieber CC, et al. Distribution but not amount of protein intake is associated with frailty: a cross-sectional investigation in the region of Nürnberg. Nutr J. 2013;12:109. https://doi.org/10.1186/1475-2891-12-109
46. Laake P, Benestad HB, Olsen BR, Brady MM. Research methodology in the medical and biological sciences. Amsterdam: Elsevier, Academic Press; 2007.
47. Otsuka R, Tange C, Tomida M, Nishita Y, Kato Y, Yuki A, et al. Dietary Factors Associated with the Development of Physical Frailty in Community-Dwelling Older Adults. J Nutr Health Aging. 2019;23(1):89-95. https://doi.org/10.1007/s12603-018-1124-3
48. Twisk JWR, Kemper HG, Van Mechelen W, Post GB. Tracking of Risk Factors for Coronary Heart Disease over a 14-Year Period: A Comparison Between Lifestyle and Biologic Risk Factors with Data from the Amsterdam Growth and Health Study. Am J Epidemiol. 1997;145(10):888-98. https://doi.org/10.1093/oxfordjournals.aje.a009048
49. Twisk JWR. Applied Longitudinal Data Analysis for Epidemiology : A Practical Guide. Cambridge: Cambridge University Press; 2003. https://doi.org/10.1017/CBO9781139342834
50. Twisk JWR. The problem of evaluating the magnitude of tracking coefficients. Eur J Epidemiol. 2003;18(11):1025-6. https://doi.org/10.1023/a:1026161919170
51. Langhammer A, Krokstad S, Romundstad P, Heggland J, Holmen J. The HUNT study: participation is associated with survival and depends on socioeconomic status, diseases and symptoms. BMC Med Res Methodol. 2012;12:143. http://dx.doi.org/10.1186/1471-2288-12-143
52. O’Caoimh R, Sezgin D, O’Donovan MR, Molloy DW, Clegg A, Rockwood K, et al. Prevalence of frailty in 62 countries across the world: a systematic review and meta-analysis of population-level studies. Age Ageing. 2021;50(1):96-104. https://doi.org/10.1093/ageing/afaa219
53. O’Caoimh R, Galluzzo L, Rodríguez-Laso Á, Van der Heyden J, Ranhoff AH, Lamprini-Koula M, et al. Prevalence of frailty at population level in European ADVANTAGE Joint Action Member States: a systematic review and meta-analysis. Ann Ist Super Sanità. 2018;54(3):226-38. https://doi.org/10.4415/ann_18_03_10
54. Langholz PL, Strand BH, Cook S, Hopstock LA. Frailty phenotype and its association with all-cause mortality in community-dwelling Norwegian women and men aged 70 years and older: The Tromsø Study 2001-2016. Geriatr Gerontol Int. 2018;18(8):1200-5. https://doi.org/10.1111/ggi.13447
55. Strand BH, Bergland A, Jørgensen L, Schirmer H, Emaus N, Cooper R. Do More Recent Born Generations of Older Adults Have Stronger Grip? A Comparison of Three Cohorts of 66- to 84-Year Olds in the Tromsø Study. J Gerontol A Biol Sci Med Sci. 2019;74(4):528-33. https://doi.org/10.1093/gerona/gly234
56. Hruby A, Jacques PF. Protein Intake and Human Health: Implications of Units of Protein Intake. Adv Nutr. 2021;12(1):71-88. https://doi.org/10.1093/advances/nmaa097
57. Johansson L, Solvoll K. Norkost 1993-94 og 1997 : Landsomfattende kostholdsundersøkelser blant menn og kvinner i alderen 16-79 år. (National dietary surveys among men and women aged 16-79 years). Rapport nr. 3/1999. Oslo: Statens råd for ernæring og fysisk aktivitet; 1999.
58. Carlsen MH, Lillegaard ITL, Karlsen A, Blomhoff R, Drevon CA, Andersen LF. Evaluation of energy and dietary intake estimates from a food frequency questionnaire using independent energy expenditure measurement and weighed food records. Nutr J. 2010;9. https://dx.doi.org/10.1186%2F1475-2891-9-37
59. Carlsen MH, Karlsen A, Lillegaard ITL, Gran JM, Drevon CA, Blomhoff R, et al. Relative validity of fruit and vegetable intake estimated from an FFQ, using carotenoid and flavonoid biomarkers and the method of triads. Br J Nutr. 2011;105(10):1530-8. https://doi.org/10.1017/S0007114510005246
60. Carlsen MH, Blomhoff R, Andersen LF. Intakes of culinary herbs and spices from a food frequency questionnaire evaluated against 28-days estimated records. Nutr J. 2011;10. https://dx.doi.org/10.1186%2F1475-2891-10-50